New Content From Advances in Methods and Practices in Psychological Science
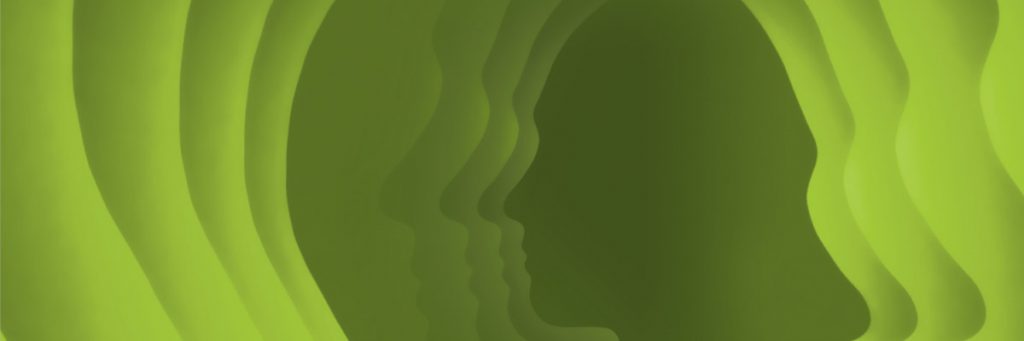
Natural Experiments: Missed Opportunities for Causal Inference in Psychology
Michael P. Grosz, Adam Ayaita, Ruben C. Arslan, et al.
Knowledge about causal effects is essential for building useful theories and designing effective interventions. The preferred design for learning about causal effects is randomized experiments (i.e., studies in which the researchers randomly assign units to treatment and control conditions). However, randomized experiments are often unethical or unfeasible. On the other hand, observational studies are usually feasible but lack the random assignment that renders randomized experiments causally informative. Natural experiments can sometimes offer unique opportunities for dealing with this dilemma, allowing causal inference on the basis of events that are not controlled by researchers but that nevertheless establish random or as-if random assignment to treatment and control conditions. Yet psychological researchers have rarely exploited natural experiments. To remedy this shortage, we describe three main types of studies exploiting natural experiments (standard natural experiments, instrumental-variable designs, and regression-discontinuity designs) and provide examples from psychology and economics to illustrate how natural experiments can be harnessed. Natural experiments are challenging to find, provide information about only specific causal effects, and involve assumptions that are difficult to validate empirically. Nevertheless, we argue that natural experiments provide valuable causal-inference opportunities that have not yet been sufficiently exploited by psychologists.
Tempered Expectations: A Tutorial for Calculating and Interpreting Prediction Intervals in the Context of Replications
Jeffrey R. Spence, David J. Stanley
Over the last decade, replication research in the psychological sciences has become more visible. One way that replication research can be conducted is to compare the results of the replication study with the original study to look for consistency, that is to say, to evaluate whether the original study is “replicable.” Unfortunately, many popular and readily accessible methods for ascertaining replicability, such as comparing significance levels across studies or eyeballing confidence intervals, are generally ill suited to the task of comparing results across studies. To address this issue, we present the prediction interval as a statistic that is effective for determining whether a replication study is inconsistent with the original study. We review the statistical rationale for prediction intervals, demonstrate hand calculations, and provide a walkthrough using an R package for obtaining prediction intervals for means, d values, and correlations. To aid the effective adoption of prediction intervals, we provide guidance on the correct interpretation of results when using prediction intervals in replication research.
Interacting With Curves: How to Validly Test and Probe Interactions in the Real (Nonlinear) World
Uri Simonsohn
Hypotheses involving interactions in which one variable modifies the association between another two are very common. They are typically tested relying on models that assume effects are linear, for example, with a regression like y = a + b x + c z + d x × z. In the real world, however, few effects are linear, invalidating inferences about interactions. For instance, in realistic situations, the false-positive rate can be 100% for detecting an interaction, and a probed interaction can reliably produce estimated effects of the wrong sign. In this article, I propose a revised toolbox for studying interactions in a curvilinear-robust manner, giving correct answers “even” when effects are not linear. It is applicable to most study designs and produces results that are analogous to those of current—often invalid—practices. The presentation combines statistical intuition, demonstrations with published results, and simulations.
Performing Small-Telescopes Analysis by Resampling: Empirically Constructing Confidence Intervals and Estimating Statistical Power for Measures of Effect Size
Samantha Costigan, John Ruscio, Jarret T. Crawford
When new data are collected to check the findings of an original study, it can be challenging to evaluate replication results. The small-telescopes method is designed to assess not only whether the effect observed in the replication study is statistically significant but also whether this effect is large enough to have been detected in the original study. Unless both criteria are met, the replication either fails to support the original findings or the results are mixed. When implemented in the conventional manner, this small-telescopes method can be impractical or impossible to conduct, and doing so often requires parametric assumptions that may not be satisfied. We present an empirical approach that can be used for a variety of study designs and data-analytic techniques. The empirical approach to the small-telescopes method is intended to extend its reach as a tool for addressing the replication crisis by evaluating findings in psychological science and beyond. In the present tutorial, we demonstrate this approach using a Shiny app and R code and included an analysis of most studies (95%) replicated as part of the Open Science Collaboration’s Reproducibility Project in Psychology. In addition to its versatility, simulations demonstrate the accuracy and precision of the empirical approach to implementing small-telescopes analysis.
Diagnosing the Misuse of the Bayes Factor in Applied Research
Jorge N. Tendeiro, Henk A. L. Kiers, Rink Hoekstra, Tsz Keung Wong, Richard D. Morey
Hypothesis testing is often used for inference in the social sciences. In particular, null hypothesis significance testing (NHST) and its p value have been ubiquitous in published research for decades. Much more recently, null hypothesis Bayesian testing (NHBT) and its Bayes factor have also started to become more commonplace in applied research. Following preliminary work by Wong and colleagues, we investigated how, and to what extent, researchers misapply the Bayes factor in applied psychological research by means of a literature study. Based on a final sample of 167 articles, our results indicate that, not unlike NHST and the [Formula: see text] value, the use of NHBT and the Bayes factor also shows signs of misconceptions. We consider the root causes of the identified problems and provide suggestions to improve the current state of affairs. This article is aimed to assist researchers in drawing the best inferences possible while using NHBT and the Bayes factor in applied research.
Careless Responding: Why Many Findings Are Spurious or Spuriously Inflated
Morgan D. Stosic, Brett A. Murphy, Fred Duong, Amber A. Fultz, Summer E. Harvey, Frank Bernieri
Contrary to long-standing conventional wisdom, failing to exclude data from carelessly responding participants on questionnaires or behavioral tasks will frequently result in false-positive or spuriously inflated findings. Despite prior publications demonstrating this disturbing statistical confound, it continues to be widely underappreciated by most psychologists, including highly experienced journal editors. In this article, we aim to comprehensively explain and demonstrate the severity and widespread prevalence of careless responding’s (CR) inflationary effects in psychological research. We first describe when and why one can expect to observe the inflationary effect of unremoved CR data in a manner accessible to early graduate or advanced undergraduate students. To this end, we provide an online simulator tool and instructional videos for use in classrooms. We then illustrate realistic magnitudes of the severity of unremoved CR data by presenting novel reanalyses of data sets from three high-profile articles: We found that many of their published effects would have been meaningfully, sometimes dramatically, inflated if they had not rigorously screened out CR data. To demonstrate the frequency with which researchers fail to adequately screen for CR, we then conduct a systematic review of CR screening procedures in studies using paid online samples (e.g., MTurk) published across two prominent psychological-science journals. These findings suggest that most researchers either did not conduct any kind of CR screening or conducted only bare minimal screening. To help researchers avoid publishing spuriously inflated findings, we summarize best practices to help mitigate the threats of CR data.
A Tutorial on Analyzing Ecological Momentary Assessment Data in Psychological Research With Bayesian (Generalized) Mixed-Effects Models
Jonas Dora, Connor J. McCabe, Caspar J. van Lissa, Katie Witkiewitz, Kevin M. King
In this tutorial, we introduce the reader to analyzing ecological momentary assessment (EMA) data as applied in psychological sciences with the use of Bayesian (generalized) linear mixed-effects models. We discuss practical advantages of the Bayesian approach over frequentist methods and conceptual differences. We demonstrate how Bayesian statistics can help EMA researchers to (a) incorporate prior knowledge and beliefs in analyses, (b) fit models with a large variety of outcome distributions that reflect likely data-generating processes, (c) quantify the uncertainty of effect-size estimates, and (d) quantify the evidence for or against an informative hypothesis. We present a workflow for Bayesian analyses and provide illustrative examples based on EMA data, which we analyze using (generalized) linear mixed-effects models to test whether daily self-control demands predict three different alcohol outcomes. All examples are reproducible, and data and code are available at https://osf.io/rh2sw/ . Having worked through this tutorial, readers should be able to adopt a Bayesian workflow to their own analysis of EMA data.
The Causal Cookbook: Recipes for Propensity Scores, G-Computation, and Doubly Robust Standardization
Arthur Chatton, Julia M. Rohrer
Recent developments in the causal-inference literature have renewed psychologists’ interest in how to improve causal conclusions based on observational data. A lot of the recent writing has focused on concerns of causal identification (under which conditions is it, in principle, possible to recover causal effects?); in this primer, we turn to causal estimation (how do researchers actually turn the data into an effect estimate?) and modern approaches to it that are commonly used in epidemiology. First, we explain how causal estimands can be defined rigorously with the help of the potential-outcomes framework, and we highlight four crucial assumptions necessary for causal inference to succeed (exchangeability, positivity, consistency, and noninterference). Next, we present three types of approaches to causal estimation and compare their strengths and weaknesses: propensity-score methods (in which the independent variable is modeled as a function of controls), g-computation methods (in which the dependent variable is modeled as a function of both controls and the independent variable), and doubly robust estimators (which combine models for both independent and dependent variables). A companion R Notebook is available at github.com/ArthurChatton/CausalCookbook. We hope that this nontechnical introduction not only helps psychologists and other social scientists expand their causal toolbox but also facilitates communication across disciplinary boundaries when it comes to causal inference, a research goal common to all fields of research.
Effect sizes are often used in psychology because they are crucial when determining the required sample size of a study and when interpreting the implications of a result. Recently, researchers have been encouraged to contextualize their effect sizes and determine what the smallest effect size is that yields theoretical or practical implications, also known as the “smallest effect size of interest” (SESOI). Having a SESOI will allow researchers to have more specific hypotheses, such as whether their findings are truly meaningful (i.e., minimum-effect testing) or whether no meaningful effect exists (i.e., equivalence testing). These types of hypotheses should be reflected in power analyses to accurately determine the required sample size. Through a confidence-interval-focused approach and simulations, I show how to conduct power analyses for minimum-effect and equivalence testing. Moreover, I show that conducting a power analysis for the SESOI might result in inconclusive results. This confidence-interval-focused simulation-based power analysis can be easily adopted to different types of research areas and designs. Last, I provide recommendations on how to conduct such simulation-based power analyses.
Capturing the Social Life of a Person by Integrating Experience-Sampling Methodology and Personal-Social-Network Assessments
Marie Stadel, Laura F. Bringmann, Gert Stulp, et al.
The daily social life of a person can be captured with different methodologies. Two methods that are especially promising are personal-social-network (PSN) data collection and experience-sampling methodology (ESM). Whereas PSN data collections ask participants to provide information on their social relationships and broader social environment, ESM studies collect intensive longitudinal data on social interactions in daily life using multiple short surveys per day. In combination, the two methods enable detailed insights into someone’s social life, including information on interactions with specific interaction partners from the personal network. Despite many potential uses of such data integration, there are few studies to date using the two methods in conjunction. This is likely due to their complexity and lack of software that allows capturing the full social life of someone while keeping the burden for participants and researchers sufficiently low. In this article, we report on the development of methodology and software for an ESM/PSN integration within the established ESM tool m-Path. We describe results of a first study using the developed tool that illustrate the feasibility of the proposed method combination and show that participants consider the assessments insightful. We further outline study-design choices and ethical considerations when combining the two methodologies. We hope to encourage applications of the presented methods in research and practice across different fields.
The Incremental Propensity Score Approach for Diversity Science
Wen Wei Loh, Dongning Ren
Addressing core questions in diversity science requires quantifying causal effects (e.g., what drives social inequities and how to reduce them). Conventional approaches target the average causal effect (ACE), but ACE-based analyses suffer from limitations that undermine their relevance for diversity science. In this article, we introduce a novel alternative from the causal inference literature: the so-called incremental propensity score (IPS). First, we explain why the IPS is well suited for investigating core queries in diversity science. Unlike the ACE, the IPS does not demand conceptualizing unrealistic counterfactual scenarios in which everyone in the population is uniformly exposed versus unexposed to a causal factor. Instead, the IPS focuses on the effect of hypothetically shifting individuals’ chances of being exposed along a continuum. This allows seeing how the effect may be graded, offering a more realistic and policy-relevant quantification of the causal effect than a single ACE estimate. Moreover, the IPS does not require the positivity assumption, a necessary condition for estimating the ACE but which rarely holds in practice. Next, to broaden accessibility, we provide a step-by-step guide on estimating the IPS using R, a free and popular software. Finally, we illustrate the IPS using two real-world examples. The current article contributes to the methodological advancement in diversity science and offers researchers a more realistic, relevant, and meaningful approach.
Practices in Data-Quality Evaluation: A Large-Scale Review of Online Survey Studies Published in 2022
Jaroslav Gottfried
In this study, I examine data-quality evaluation methods in online surveys and their frequency of use. Drawing from survey-methodology literature, I identified 11 distinct assessment categories and analyzed their prevalence across 3,298 articles published in 2022 from 200 psychology journals in the Web of Science Master Journal List. These English-language articles employed original data from self-administered online questionnaires. Strikingly, 55% of articles opted not to employ any data-quality evaluation, and 24% employed only one method despite the wide repertoire of methods available. The most common data-quality indicators were attention-control items (22%) and nonresponse rates (13%). Strict and unjustified nonresponse-based data-exclusion criteria were often observed. The results highlight a trend of inadequate quality control in online survey research, leaving results vulnerable to biases from automated response bots or respondents’ carelessness and fatigue. More thorough data-quality assurance is currently needed for online surveys.
Implementing Statcheck During Peer Review Is Related to a Steep Decline in Statistical-Reporting Inconsistencies
Michèle B. Nuijten, Jelte M. Wicherts
We investigated whether statistical-reporting inconsistencies could be avoided if journals implement the tool statcheck in the peer-review process. In a preregistered pretest–posttest quasi-experiment covering more than 7,000 articles and more than 147,000 extracted statistics, we compared the prevalence of reported p values that were inconsistent with their degrees of freedom and test statistics in two journals that implemented statcheck in their peer-review process (Psychological Science and Journal of Experimental and Social Psychology) and two matched control journals (Journal of Experimental Psychology: General and Journal of Personality and Social Psychology) before and after statcheck was implemented. Preregistered multilevel logistic regression analyses showed that the decrease in both inconsistencies and decision inconsistencies around p = .05 is considerably steeper in statcheck journals than in control journals, offering preliminary support for the notion that statcheck can be a useful tool for journals to avoid statistical-reporting inconsistencies in published articles. We discuss limitations and implications of these findings.
Listen to related podcast episode.
Feedback on this article? Email [email protected] or login to comment.
APS regularly opens certain online articles for discussion on our website. Effective February 2021, you must be a logged-in APS member to post comments. By posting a comment, you agree to our Community Guidelines and the display of your profile information, including your name and affiliation. Any opinions, findings, conclusions, or recommendations present in article comments are those of the writers and do not necessarily reflect the views of APS or the article’s author. For more information, please see our Community Guidelines.
Please login with your APS account to comment.