How Wearable Device Data Can Fuel Digital Interventions
Researchers are using data from wearable devices to deliver digital interventions when people need them most.
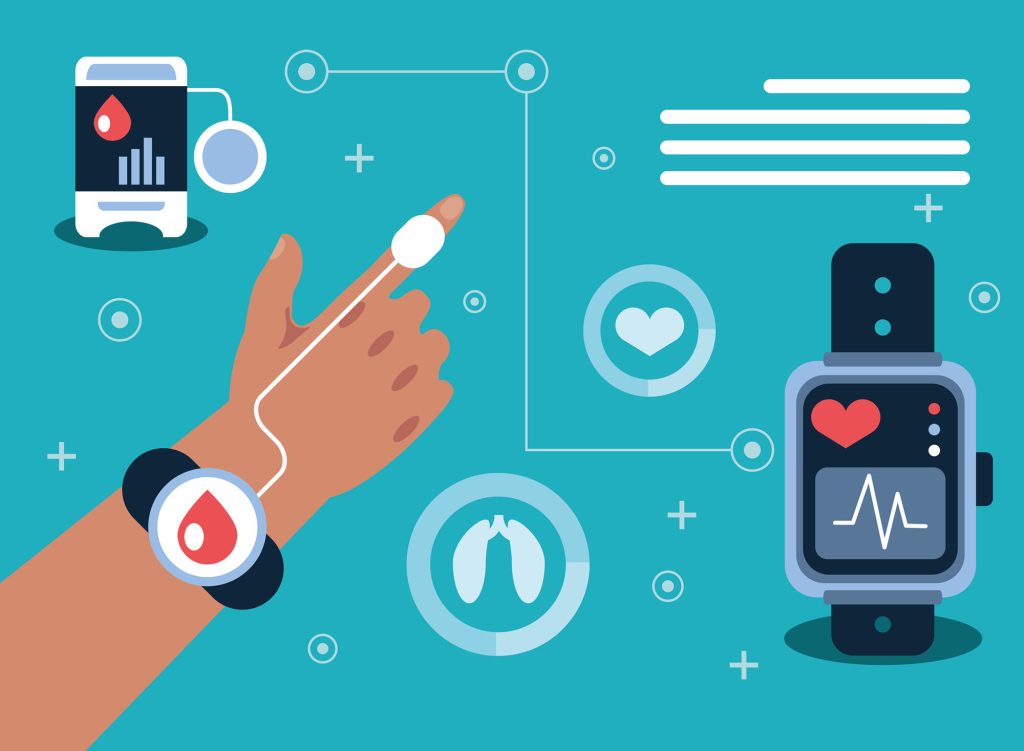
- Digital interventions such as mood tracking and smoking cessation apps, informed by wearable device data, can support treatment for mental health conditions by helping patients use effective emotion-regulation strategies in their daily lives.
- Digital interventions can help users overcome barriers to mental health treatment, including cost, insurance coverage, transportation, the limited availability of providers, and stigma against openly receiving treatment.
- Understanding the physical, cognitive, and emotional components of engagement with wearable devices could help developers identify the level of engagement at which an intervention delivers the most positive behavior change while demanding as little of participants’ time and effort as possible.
- Declining engagement doesn’t necessarily mean a digital intervention is ineffective—it could also signal that a user has learned to use a behavioral strategy without continuing to interact with an app.
- Hybrid experimental design allows researchers to assess the effectiveness of interventions that operate on multiple timescales, including digital just-in-time interventions and human-delivered therapies.
Understanding engagement as a process • Measuring multimodal interventions
Most of us go about our days surrounded by devices that can passively monitor our behavior. In addition to the computer, phone, or tablet you’re using to read this article, you may have a smartwatch for tracking your activity level. The truly tech-savvy may adorn themselves with smart rings or glasses and dream of the day when brain chips hit the open market.
These and other wearable devices, including skin patches and other unobtrusive monitors designed to measure glucose levels and heart activity in medical settings, provide an unprecedented window into the moment-to-moment relationship between our physical and mental health. By leveraging this treasure trove of information, researchers have already begun providing the public with new tools to support behavior change.
Related Content: Teaching: Wearable Cognitive Assistants
“Technology—which increasingly mediates and supports human activities—retains digital traces of people’s behaviors, creating a gold mine of data for psychological science,” wrote behavioral scientist Anat Rafaeli and colleagues at the Israel Institute of Technology in a Current Directions in Psychological Science article. Unlike experiments, surveys, and diary studies, which often rely on brief periods of observation or participants’ retrospective reports of their own behavior, wearable devices can meticulously document people’s daily behaviors and emotions as they happen, as well as the contexts in which they occur, Rafaeli and colleagues explained (Rafaeli et al., 2019).
This wealth of information can also help mental health practitioners fill in the “clinical whitespace” between appointments with their patients, said Glen Coppersmith, chief data officer at the therapy company SonderMind, in a 2023 interview with the Observer. Using wearables and apps to track patients’ well-being over time can uncover patterns that patients may not be able to report themselves and help therapists provide more effective, measurement-based treatment recommendations, he said.
“Digital technologies are a central feature of daily life for many children, adolescents, and adults, and these technologies afford researchers the opportunity to examine a wide range of emotion-regulation processes as they unfold in real time,” a team of clinical psychologists wrote in a Clinical Psychological Science article. The authors included Alexandra H. Bettis (Vanderbilt University Medical Center), Taylor A. Burke and Jacqueline Nesi (Brown Medical School), and APS Fellow Richard T. Liu (Harvard Medical School).
Ecological momentary assessment (EMA)—using real-time data collected from smartphones paired with wearables such as watches, bracelets, and patches—could also help people regulate their emotions in the moment, Bettis and colleagues wrote. Mobile sensors that can measure autonomic nervous system activity based on a person’s heart rate and breathing may be particularly valuable when it comes to identifying not only if a person is stressed, but if the emotion-regulation strategies they are using are effective, the researchers noted. Individuals’ location data can also be used to identify when a person is entering an area associated with certain symptoms or risky behaviors, allowing their device to prompt them to employ appropriate coping strategies. These data can also be compared with individuals’ self-reported symptoms and mood through diary apps to gain further insight into their well-being.
Providing patients with apps designed to deliver ecological momentary interventions, in addition to therapy, has been used to successfully support the treatment of anxiety, social anxiety, insomnia, schizophrenia, and substance use, the researchers noted. This dual approach to treatment can allow patients to benefit from both human-delivered therapy sessions and digital just-in-time adaptive interventions tailored to their specific needs, Bettis and colleagues wrote.
Digital interventions informed by data from wearable devices could help address many of the barriers to mental health treatment, including cost, lack of public transportation, the limited availability of providers, and stigma against openly pursuing mental health treatment, the authors added.
“New models of mental health treatment are needed that move beyond traditional weekly psychotherapy,” they wrote. “These digital tools could radically change the mental health service landscape” (Bettis et al., 2021).
Understanding engagement as a process
To make full use of the data collected by these devices, researchers need to understand how people engage with digital interventions, which requires framing engagement as an ongoing process, behavioral researcher Inbal Nahum-Shani and APS Fellow Carolyn Yoon (University of Michigan) wrote in a recent article for Current Directions in Psychological Science.
Nahum-Shani and Yoon’s model frames this engagement as a multi-faceted process involving physical, cognitive, and emotional energy directed at a collection of interrelated digital and nondigital stimuli (e.g., notifications and reminders) and tasks, such as opening an app or practicing a behavior.
“By developing a pragmatic process model of engagement with digital interventions that reflects this nuanced understanding, we can achieve more accurate measurements of engagement and inform the development of more effective strategies,” Yoon said in an interview with the Observer.
This perspective could help researchers identify what constitutes “optimal engagement” with an intervention, including the frequency, duration, and nature of use needed to balance effectiveness with efficiency, Nahum-Shani and Yoon explained. Ideally, the goal is to identify the level of engagement at which an intervention delivers the greatest positive behavior change while demanding as little of participants time and effort as possible (Nahum-Shani & Yoon, 2024).
To meet that goal, researchers need to articulate how they expect participants to engage with an intervention so that they know what to study, the authors continued.
“Developers of wearable devices may find it useful to create pragmatic models of engagement that map out how users are expected to engage with different device features, stimuli, and tasks over time,” Yoon said.
These maps might include real-life barriers to using an intervention, such as the need to charge a device, the researchers note. Putting these maps to use may also require new methods for tracking users’ engagement with both digital content and real-life tasks, and greater understanding of how that engagement may shift over time.
“Declining engagement with a digital intervention may not necessarily signify its ineffectiveness. Rather, it may indicate that certain digital stimuli or tasks are not needed for downstream nondigital tasks,” Nahum-Shani and Yoon wrote. For example, if an individual is using a mindfulness app to help them stop smoking, they may initially start out opening the app in response to a notification that prompts them to engage in a breathing exercise to reduce cravings. Over time, they may stop opening the app in response to the notification as they learn to complete the exercise on their own.
“Viewing engagement as an ongoing process rather than a fixed state could influence how wearable-device developers think about user retention,” Yoon said. “It might lead to more dynamic, adaptive interfaces and features that evolve based on the user’s changing engagement patterns over time.”
Developers may be able to increase engagement by identifying the moments in which users are most receptive to digital interventions using artificial intelligence and machine learning, Yoon suggested. This could be done by altering the timing or content of notifications.
This perspective on digital interventions could help improve user outcomes by providing wearable-device developers with a deeper understanding of how they are being used, Yoon said.
“The devices could become more effective, user-friendly, and personalized. They would better support positive behavior change and outcomes for users in the short term and the long term,” Yoon said.
Measuring multimodal interventions
Interventions that incorporate digital technology and human practitioners could also help boost engagement while cutting expenses, according to an article Nahum-Shani coauthored for Advances in Methods and Practices in Psychological Science.
“The integration of digital and human-delivered components is critical to building effective and scalable psychological interventions,” Nahum-Shani and colleagues wrote. When these components are integrated synergistically, it can lead to the creation of interventions that are greater than the sum of their parts, the researchers continued. Researchers will need to use a new approach to experimental design to investigate how best to construct interventions that operate on multiple timescales.
Digital and human-delivered interventions, also known as multimodality adaptive interventions (MADIs), consist of two parts, Nahum-Shani and her colleagues explained. Digital just-in-time adaptive interventions (JITAIs) can offer people motivational messages and suggest behavioral strategies in response to minute-to-minute changes in their mood and location, among other factors. Human-delivered adaptive interventions (ADIs), on the other hand, are generally offered to a person by a health practitioner during weekly or monthly appointments in response to their progress on achieving long-term goals.
Nahum-Shani and colleagues’ new experimental approach, called hybrid experimental design (HED) allows researchers to assess the effectiveness of MADIs by providing a method for randomly assigning participants to different conditions at both minute-to-minute and long-term levels. This experimental design can provide researchers with valuable insight into how to combine and sequence multimodal interventions.
To illustrate their methodology, the scientists presented a hypothetical intervention designed to reduce drug use and violent behavior among adolescents after admission to an emergency room. In this multimodal approach, participants would receive an in-person information session about risk reduction and resilience from a practitioner in the ER and then be given access to an app that delivers daily messages about behavioral strategies for avoiding drug use in response to self-reported stress and loneliness. Participants who don’t respond to this initial intervention after 4 weeks would then be offered a telehealth session with a practitioner for further behavioral coaching.
Scientific questions about how to best construct this MADI can be answered with a HED where participants are randomly assigned at treatment outset and week 4 to different intensities of telehealth coaching, and at the same time they also get randomly assigned every day to different types of messages, Nahum-Shani explained.
This and other methodological advances are taking researchers a step closer to identifying the crucial moments in which individuals are most receptive to behavioral interventions, empowering people to transform their abundant data into an abundance of well-being.
Feedback on this article? Email [email protected] or login to comment.
Related content we think you’ll enjoy
-
The Pluses and Pitfalls of Online Research
Online platforms like MTurk and Prolific have dramatically broadened scientists’ access to study participants. But are researchers using the tools effectively?
-
The Bad-Behavior Blend
“The one gene, one disease idea is a thing of the past.” Scientists aren’t simply trying to identify people who are innately predisposed to incivility, immorality, or lawlessness. They’re examining how parenting, education, and other life experiences trigger those biological leanings.
-
Childhood Adversity
Psychological research reveals the long-lasting cognitive, social, and neurobiological consequences of stress and trauma experienced in childhood.
References
Bettis, A. H., Burke, T. A., Nesi, J., & Liu, R. T. (2022). Digital technologies for emotion-regulation assessment and intervention: A conceptual review. Clinical Psychological Science, 10(1), 3–26. https://doi.org/10.1177/21677026211011982
Nahum-Shani, I., Dziak, J. J., Walton, M. A., & Dempsey, W. (2022). Hybrid experimental designs for intervention development: What, why, and how. Advances in Methods and Practices in Psychological Science, 5(3). https://doi.org/10.1177/25152459221114279
Nahum-Shani, I., & Yoon, C. (2024). Toward the science of engagement with digital interventions. Current Directions in Psychological Science. https://doi.org/10.1177/09637214241254328
Rafaeli, A., Ashtar, S., & Altman, D. (2019). Digital traces: New data, resources, and tools for psychological-science research. Current Directions in Psychological Science, 28(6), 560–566. https://doi.org/10.1177/0963721419861410
APS regularly opens certain online articles for discussion on our website. Effective February 2021, you must be a logged-in APS member to post comments. By posting a comment, you agree to our Community Guidelines and the display of your profile information, including your name and affiliation. Any opinions, findings, conclusions, or recommendations present in article comments are those of the writers and do not necessarily reflect the views of APS or the article’s author. For more information, please see our Community Guidelines.
Please login with your APS account to comment.