Tailoring Evidence-Based Treatment to the Person, Not the Diagnosis
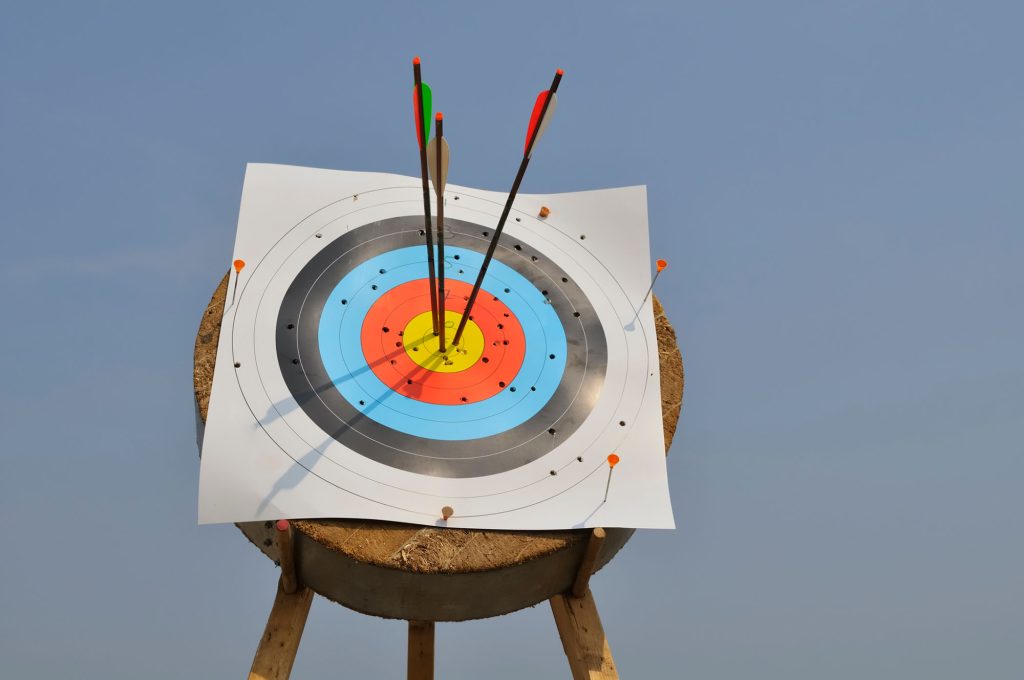
- Many evidence-based treatments are based on averages and don’t work for every patient.
- Major granting institutions support the concept of personally tailored treatment.
- Clinical psychologist Cheri Levinson is among researchers developing a form of precision treatment that targets specific symptoms that an individual presents.
- Levinson hopes her model will expand the effective use of evidence-based treatments in clinical settings.
Historically, mental healthcare providers have viewed evidence-based treatments with a mix of frustration and skepticism. Some find that even the most scientifically supported interventions for depression or anxiety, for example, don’t work for every patient.
“Clinicians may draw from a manual or from different evidence-based treatments, but they don’t necessarily like to follow the manual step by step because their patient is often very different than the on-average patient,” said Cheri Levinson, a clinical psychology researcher at the University of Louisville.
Read this 2022 Back Page profile on Cheri Levinson and the devastating toll of diet culture.
Levinson is in the forefront of developing scientifically sound treatments designed for the person, not the diagnosis. In an article published in Clinical Psychological Science, Levinson and her colleagues propose that analysis of an individual patient’s data, with the aid of new technologies, can help get more evidence-based treatments into practice.
These transdiagnostic personalized treatments won’t be a “magic bullet,” Levinson and her coauthors state, noting that reducing the burden of mental illness will require a combination of many solutions. But the new model stands to advance the application of science-backed treatments, she added.
“This actually has the potential to make treatments more effective—to actually get used by clinicians instead of developing these treatments that then never reach the intended audience,” Levinson said in an interview.
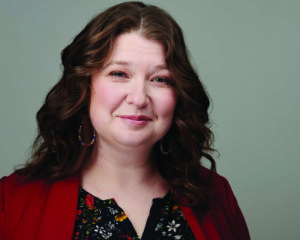
Why the resistance?
Several factors account for practitioners’ hesitancy to adopt evidence-based interventions, Levinson and her colleagues—University of Louisville graduate student Caroline Christian and APS Fellow Carolyn Becker of Trinity University—wrote in their paper. For one, many treatments are manualized and focus on a specific disorder or problem, even though most patients meet the criteria for more than one disorder, they explained.
“Even if clinicians are committed to using evidence-based interventions, there is scant research guiding them on which disorder (or primary problem) to treat first … which forces a reliance on flawed clinical judgments,” they wrote.
Many interventions are created from findings that apply to a population of patients rather than individuals, Levinson added.
“We can say, for example, that exposure therapy or CBT [cognitive behavioral therapy] works on average for most people who have social anxiety disorder,” Levinson told APS. “And that’s a really important approach because it helps us figure out what works for many people. But it doesn’t account for the fact that huge groups of people don’t respond to those treatments.”
Major granting institutions, including the National Institute of Mental Health (NIMH) in the United States, support the concept of personally tailored treatment, citing research showing that it produces long-lasting and effective outcomes. And Levinson is among early-career scientists eager to get those treatments into practice.
The cornerstone of Levinson’s work is network theory. Proposed by Denny Borsboom (University of Amsterdam), network theory states that mental disorders arise from a set of symptoms that reinforce each other and create a kind of feedback loop (Borsboom, 2017). A patient presenting with insomnia, for example, becomes fatigued, which leads to low mood, a lack of appetite, and weight loss, which then leads to an eating disorder that worsens the other symptoms.
In what is referred to as idiographic network modeling, a patient’s thoughts, behaviors, and emotions become a dataset that helps illuminate symptom connections at the individual level. The data can be collected at multiple time points through experience sampling methods delivered on mobile phones or tablets.
Idiographic network analysis can be applied within the context of existing evidence-based treatments, such as CBT and dialectic behavior therapy, Levinson and her coauthors wrote. Clinicians can use it to decide which parts of those protocols should be used when and for whom, they added.
“Instead of saying, ‘This person has social anxiety disorder and I’m going to apply CBT,’ you would say this person is fearful of social situations where there’s more than 10 people and they are afraid of being judged,” Levinson explained. “Then you would specifically look at interventions that are targeted at those two fears and address those instead of just overall broad CBT.”
Success in trials
Levinson and her team have created a treatment called transdiagnostic network-informed personalized treatment (T-NIPT) and have been testing it as a treatment for eating disorders. Using patient data collected via smartphone, T-NIPT identifies symptoms to target with existing evidence-based treatments.
A patient could be assessed at intake but also log symptoms over a smartphone for 15 days. That smartphone data is automatically processed through software and creates an idiographic network for the patient. Symptoms are identified and matched to evidence-based treatments that the clinician can employ.
Levinson’s team found that T-NIPT had strong clinical efficacy, decreasing impairment, worry, and other symptoms related to eating disorders (Levinson, 2023).
This personalized approach to treating eating disorders has earned enthusiastic attention from grantors. In 2023, she was named one of the National Institute of Health Director’s Innovators of the Year.
T-NIPT needs plenty more research but shows promise for the treatment of many psychiatric illnesses, Levinson said. It could lead to shorter, more effective treatments, enabling clinicians to treat more clients. It still relies on clinicians’ expertise but equips them with more data to bolster treatment effectiveness.
Levinson and her team are also exploring how to incorporate social and cultural factors into precision treatment. Poverty, racism, and other social problems can emerge as barriers to recovery, she noted.
“If a patient is experiencing food insecurity, it’s going to be really hard to work on some of those other symptoms,” she said. “How am I going to get the patient into regular eating if they can’t even afford food? We do need to think about how to integrate these systemic factors into treatment.”
Idiographic network modeling generates treatment strategies that essentially focus on symptoms that underlie a diagnosis, rather than the diagnosis itself, she said.
“If we could really figure out what these important symptoms are across psychopathology, and then figure out what are treatments that work for those specific symptoms,” she said, “we’d really go a long way to decreasing the suffering from mental illness.”
Feedback on this article? Email [email protected] or login to comment.
References
Borsboom, D. (2017). A network theory of mental disorders. World Psychiatry, 16(1), 5–13. https://doi.org/10.1002/wps.20375
Levinson, C. A., Christian, C., & Becker, C. B. (2024). How idiographic methodologies can move the clinical-science field forward to integrate personalized treatment into everyday clinical care and improve treatment outcomes. Clinical Psychological Science. https://doi.org/10.1177/21677026231217316
Levinson, C. A., Williams, B. M., Christian, C., Hunt, R. A., Keshishian, A. C., Brosof, L. C., Vanzhula, I. A., Davis, G. D., Brown, M. L., Bridges-Curry, Z., Sandoval-Araujo, L. E., & Ralph-Nearman, C. (2023). Personalizing eating disorder treatment using idiographic models: An open series trial. Journal of Consulting and Clinical Psychology, 91(1), 14–28. https://doi.org/10.1037/ccp0000785
APS regularly opens certain online articles for discussion on our website. Effective February 2021, you must be a logged-in APS member to post comments. By posting a comment, you agree to our Community Guidelines and the display of your profile information, including your name and affiliation. Any opinions, findings, conclusions, or recommendations present in article comments are those of the writers and do not necessarily reflect the views of APS or the article’s author. For more information, please see our Community Guidelines.
Please login with your APS account to comment.