When One Size Doesn’t Fit All
Uncovering individual and contextual differences in experimental outcomes
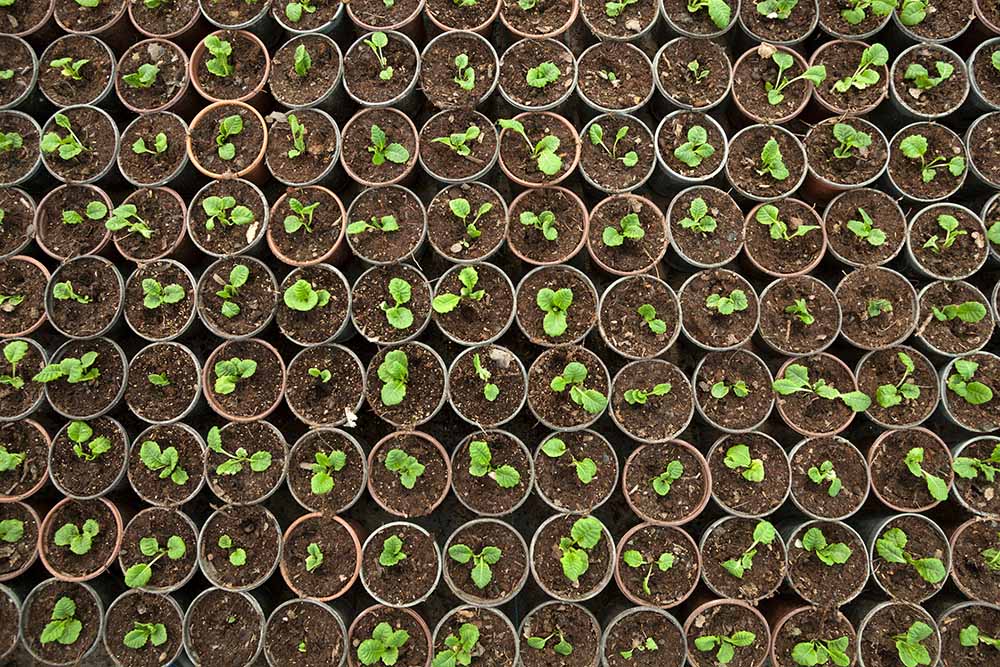
- Many social and behavioral scientists are calling for methodological approaches that examine not only the effect of a treatment or intervention but also when, where, and with whom the effect works.
- By adopting a more nuanced, context-aware approach to their research, scientists can more precisely target certain clinical treatments, behavioral and educational interventions, and policies to maximum effect.
- Some statistics experts warn researchers to temper their expectations about what new methods can realistically show about heterogeneous effects, at least in the short term.
Examining the disconnect • Capturing the individual variations • Risks of excess • Building the infrastructure
To take root and thrive, a seed must be planted in optimal soil.
Gregory M. Walton and David S. Yeager use this analogy to assert that psychological manipulations may succeed only under certain contexts. In their own work, the psychological researchers have shown how school environments (the soil) influence the success of interventions (the seeds) designed to motivate students (Walton & Yeager, 2020).
Like Walton (Stanford University) and Yeager (University of Texas at Austin), many social and behavioral scientists are calling for methodological approaches that examine not only the effect of a treatment or intervention but also when, where, and with whom the effect works.
Several methodology experts have cited the detection of these variables—generally referred to as effect heterogeneity—as the next big challenge in social and behavioral science. For too long, many experiments have collected data from large groups and assumed that the findings can be generalized to individuals or subgroups, said APS Fellow Niall Bolger, a Columbia University social psychologist and methodology instructor. But a single intervention can produce strong effects in some people, weak or no effect in others, and even reverse effects in some cases, he cautioned.
“If you look even at handbooks of experimental psychology over the decades, there’s almost nothing on individual differences in effects,” Bolger said in an interview. “They just acknowledge them and treat them as noise.”
Many psychological scientists are now calling for a “heterogeneity revolution” (Bryan et al., 2021). Embracing heterogeneity will both improve the replicability of behavioral science and make interventions more effective and equitable, they said. By shifting from a one-size-fits-all mindset to a more nuanced, context-aware approach, they predict that researchers will be able to more precisely target certain clinical treatments, behavioral and educational interventions, and policies.
Amid this growing emphasis on heterogeneity, some social scientists call for their colleagues to temper their expectations on what the heterogeneity revolution can achieve. In a forthcoming article for Advances in Methods and Practices in Psychological Science, education researchers Paul T. von Hippel (University of Texas at Austin) and Brendan Schuetze (University of Utah) noted that past efforts to identify significant interactions and moderators have yielded disappointing results.
“I think heterogeneity is really critical to understanding psychological interventions,” said Schuetze, who uses computational models to predict where, when, and how motivational interventions work for different individuals and in different school contexts. “But I think we also have to be very careful, because many researchers looking for heterogeneity are still using statistical tools that have produced unreliable findings for decades.”
Examining the disconnect
Researchers have illuminated the heterogeneity gap through meta-analyses, with some showing that empirical conclusions vary considerably depending on study design and analytical approaches. A team of social scientists in Europe, for example, examined data from 86 meta-scientific studies and concluded that a statistically significant effect in one study may not hold across different research methods (Holzmeister et al., 2024).
Meanwhile, clinical psychologist Aaron J. Fisher of the University of California, Berkeley, and his colleagues analyzed data from six studies comparing statistical estimates derived from groups to those derived from individual participants. While they found some general agreement in the average results between group-level data and individual outcomes, they also found that the variability across statistical estimates derived from individuals was 2 to 4 times greater than the variability measured in the groups. The finding, they said, suggests that group-level results often overestimate how consistent psychological and social processes are across different people (Fisher et al., 2018).
U.K. psychological researchers Audrey Helen Linden and Johannes Hönekopp examined 150 meta-analyses in cognitive, organizational, and social psychology, along with 57 close replications. They found high levels of unexplained heterogeneity in meta-analyses and moderate heterogeneity in close replications (which tightly follow previous research designs). This suggests that psychological science has yet to establish consistent, reliable findings across different research methods, they said in Perspectives in Psychological Science (Linden & Hönekopp, 2021).
Group-to-person generalizability remains largely overlooked both within and outside of scientific circles, researchers from Boston College and the University of Exeter recently concluded in a survey of more than 830 laypeople and social psychologists. Led by Ryan McManus, now a data scientist and consultant, the team found that both researchers and the general public typically assume that group-level findings represent the majority of participants. They also culled examples from the literature where group-level conclusions describe only a small subset of participants (McManus et al., 2023).
“If psychology aims to understand the mind as a property of persons—to uncover the uniqueness or universality of certain psychological processes—person-level responses ought to be the explananda,” they wrote.
Capturing the individual variations
Many methodology experts recommend a greater use of within-subjects or repeated-measures study designs to measure effect heterogeneity. In these studies, the same participants go through multiple conditions, helping researchers see how each person responds differently. By contrast, between-subjects studies compare different groups of people, but they can’t always tell whether differences are due to the experiment or to other factors like measurement errors.
Using example data from cognitive and social psychology, Bolger and his colleagues at Columbia have highlighted the ability of linear mixed models, now replacing traditional repeated-measures analyses of variance (ANOVAs), to account for heterogeneity in experimental effects. These models, he said, permit experimenters to estimate the mean and standard deviation of effects—that is, the effect size for the average individual and the extent of variation across individuals (Bolger et al., 2019).
“Although mixed models are now well-known in psychology, the new way of thinking they permit is still largely absent in mainstream experimental work,” Bolger said in an interview, “partly because it makes things more complicated. That’s because now you can’t talk about the one effect anymore. You have to talk about a whole distribution of effects.”
Psychological researchers should develop theories that explain why people respond differently to a specific intervention, he added.
McManus and his colleagues recommend other steps to ferret out within-person variability, including the following:
- Instead of reporting only mean differences between conditions, analyze and report how many individual participants exhibit the expected effect.
- Calculate and disclose the percentage of participants whose responses align with the hypothesized effect.
- Rather than just testing for statistical significance, report how widespread the effect is across participants.
- Use metrics that describe effect sizes at the individual level rather than just at the group level.
- Report person-level results alongside group-level statistics.
Risks of excess
Some researchers maintain a cautious attitude toward the focus on heterogeneity. Organizational scientists note that moderator effects in the organizational sciences tend to be small and lacking in statistical power (Murphy & Russell, 2017). In a review of clinical trials, out of 117 subgroup effects claimed in study abstracts, only five had been subjected to replication attempts, and none of those attempts successfully replicated the subgroup effect (Wallach et al., 2017).
What’s more, some massive research projects have shown moderated effects to be less replicable than main effects.
- Across four large-scale efforts to replicate published results in psychology, economics, and social science, only one in five interactions were replicated successfully versus half of main effects (Altmejd et al., 2019).
- In a massive multilab replication project, a team of 190 researchers from across the globe found that population characteristics had little to no bearing on the failure of a finding to replicate (Klein et al., 2018).
- In a meta-analysis of multilab replications in social and cognitive psychology, Tilburg University data scientists also concluded that minor changes in sample population and study settings have minimal impact on replication study outcomes (Olsson-Collentine et al., 2020).
“While attention to heterogeneity may sometimes clear up the mystery of a non-replicable effect, to date it seems that the pursuit of moderators—at least as it’s typically been conducted—may have made the replication crisis worse instead of better,” von Hippel and Schuetze wrote in their AMPPS paper.
Many previous studies claiming to find differences in experimental and treatment effects often emanate from small or unreliable datasets, they said. The conclusions are, therefore, exaggerated, false, or not reproducible, they asserted.
von Hippel and Schuetze offer a variety of specific recommendations to help scientists avoid the mistakes they’ve linked to the focus on moderators. Here are some of their main suggestions:
- Look for differences only when there’s a good reason. Seek out heterogeneity only when there’s a solid theory behind it and enough data to detect meaningful patterns.
- Avoid chasing heterogeneity without a main effect. If a treatment doesn’t work overall, it’s riskier to go digging for small groups where it might work.
- Don’t get fooled by randomness. Many observed variations are just due to random estimation error, not actual differences. Remember that estimated effects will always vary from one group to another, even if the true effect is constant across all groups.
- Use statistical corrections for multiple comparisons. When running tests across many subgroups, the chances of finding spurious differences increases, so make adjustments to avoid misleading results.
- Shrink extreme estimates toward the average. When estimates of treatment effects are noisy, use statistical techniques to adjust these values toward the overall average. This can produce more reliable and stable estimates.
Building the infrastructure
“There is some cross-cultural work suggesting that even basic vision processes can differ depending on where you grew up,” she said. “It might be true that certain processes are so basic that they’re universal—that everybody kind of has them—but you can’t say that until you actually test it.”
Maureen craig
Amid the caution, scientific fields are taking steps to better understand nuanced responses to interventions, behavioral scientist Christopher J. Bryan (University of Texas at Austin) and colleagues pointed out in a recent article. Many journal editors and funders are encouraging authors to communicate the probable limits on the generalizability of their findings. Statisticians are developing new methods for recruiting diverse samples as well as readily available, off-the-shelf machine-learning algorithms that can be used to detect and understand heterogeneous causal effects while curbing the risk of false discoveries.
Bryan, along with Yeager and Northwestern University statistician Elizabeth Tipton, point to the importance of a robust infrastructure that provides scientists access to large representative samples along with measurement and analysis of moderators. As a model, they laud the U.S.-based Time-sharing Experiments for the Social Sciences (TESS), funded by the National Science Foundation (Bryan et al., 2021). Launched at the turn of the century, TESS supports general population experiments on behalf of social scientists. TESS contracts with the National Opinion Research Center at the University of Chicago, which conducts surveys using its AmeriSpeak Panel—a nationally representative, probability-based sample.
Investigators submit experiment proposals online for peer review. Those that are approved will get their standard data collection and dissemination costs covered through TESS.
“The goal is to help social scientists do good experiments on quality samples that allow for testing of the heterogeneity of effects that they might have a harder time doing with a less diverse sample,” said Duke University social psychologist Maureen Craig, one of three principal investigators with the program.
Social psychologists tend to be acutely aware of the role of context and group identities in shaping behavior but are often less focused on individual differences, Craig said. As such, the heterogeneity revolution could be important for social psychology as well as many other areas of study.
“There is some cross-cultural work suggesting that even basic vision processes can differ depending on where you grew up,” she said. “It might be true that certain processes are so basic that they’re universal—that everybody kind of has them—but you can’t say that until you actually test it.”
Feedback on this article? Email apsobserver@psychologicalscience.org or login to comment.
Altmejd, A., Dreber, A., Forsell, E., Huber, J., Imai, T., Johannesson, M., Kirchler, M., Nave, G., & Camerer, C. (2019) Predicting the replicability of social science lab experiments. PLOS ONE, 14(12), Article e0225826.
Bolger, N., Zee, K. S., Rossignac-Milon, M., & Hassin, R. R. (2019). Causal processes in psychology are heterogeneous. Journal of Experimental Psychology: General, 148(4), 601–618.
Bryan, C. J., Tipton, E., & Yeager, D. S. (2021). Behavioural science is unlikely to change the world without a heterogeneity revolution. Nature Human Behavior, 5, 980–989.
Fisher, A. J., Medaglia, J. D., & Jeronimo, B. F. (2018). Lack of group-to-individual generalizability is a threat to human subjects research. PNAS, 115(27), E6106–E6115.
Holzmeister, F., Johannesson, M., Bohm, R., & Kirchler, M. (2024). Heterogeneity in effect size estimates. PNAS, 121(32), Article e2403490121.
Klein, R. A., Vianello, M., Hasselman, F., Adams, B. G., Adams, R. B., Jr., Alper, S., Aveyard, M., Axt, J. R., Babalola, M. T., Bahník, Š., Batra, R., Berkics, M., Bernstein, M. J., Berry, D. R., Bialobrzeska, O., Binan, E. D., Bocian, K., Brandt, M. J., Busching, R. … Nosek, B. A. (2018). Many Labs 2: Investigating variation in replicability across samples and settings. Advances in Methods and Practices in Psychological Science, 1(4), 443–490.
Linden, A. H., & Hönekopp, J. (2021). Heterogeneity of research results: A new perspective from which to assess and promote progress in psychological science. Perspectives on Psychological Science, 16(2), 358–376.
McManus, R. M., Young, L., & Sweetman, J. (2023). Psychology Is a property of persons, not averages or distributions: Confronting the group-to-person generalizability problem in experimental psychology. Advances in Methods and Practices in Psychological Science, 6(3).
Murphy, K. R., & Russell, C. J. (2017). Mend it or end it: Redirecting the search for interactions in the organizational sciences. Organizational Research Methods, 20, 549–573.
Olsson-Collentine, A., Wicherts, J. M., van Assen, M. A. L. M. (2020). Heterogeneity in direct replications in psychology and its association with effect size. Psychological Bulletin, 146(10), 922–940.
von Hippel, P. T., & Schuetze, B. A. (in press). How not to fool ourselves about heterogeneity of treatment effects. Advances in Methods and Practices in Psychological Science.
Wallach, J. D., Sullivan, P. G., Trepanowski, J. F., Sainani, K. L., Steyerberg, E. W., & Ioannidis, J. P. A. (2017). Evaluation of evidence of statistical support and corroboration of subgroup claims in randomized clinical trials. JAMA Internal Medicine, 177(4), 554–560.
Walton, G. M., & Yeager, D. S. (2020). Seed and soil: Psychological affordances in contexts help to explain where wise interventions succeed or fail. Current Directions in Psychological Science, 29(3), 219–226.
Comments
If more psychologists used nonparametric null-hypothesis tests (e.g., Mann-Whitney or Wilcoxon signed ranks), there would be more use of effect size measures like Cliff’s (1993 Psychological Bulletin) “dominance statistics” or the “probability of superiority” (see, e.g., Ruscio’s 2008 article in Psychological Methods) and the individual differences would not be hidden by the mean or median differences.
APS regularly opens certain online articles for discussion on our website. Effective February 2021, you must be a logged-in APS member to post comments. By posting a comment, you agree to our Community Guidelines and the display of your profile information, including your name and affiliation. Any opinions, findings, conclusions, or recommendations present in article comments are those of the writers and do not necessarily reflect the views of APS or the article’s author. For more information, please see our Community Guidelines.
Please login with your APS account to comment.