Insights From High-Risk Fields Can Help Minimize Mistakes in the Lab
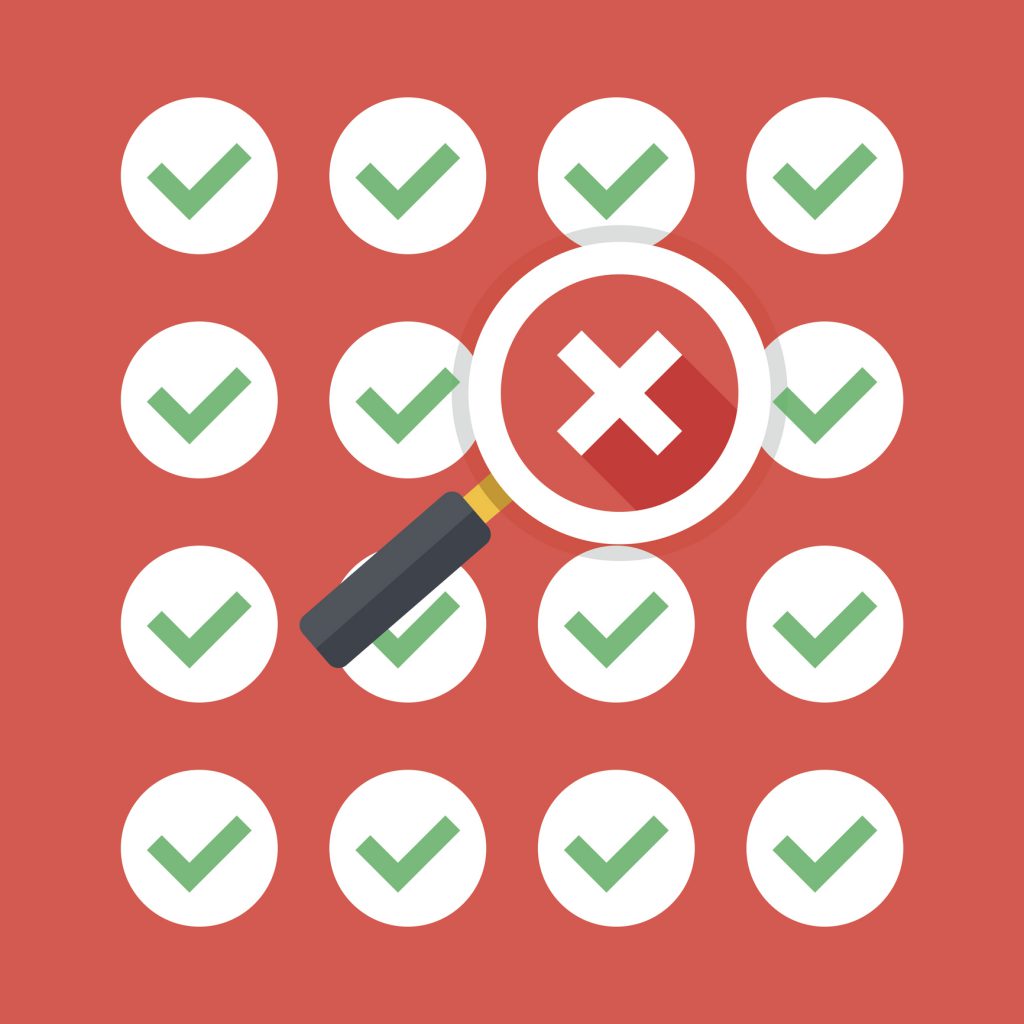
Everyone makes mistakes, including psychology researchers. Small missteps, such as typing a name incorrectly or forgetting to write down an important piece of code, can have significant and frustrating consequences for identifying research mistakes and eliminating them before a manuscript is submitted for publication. In an article published in Advances in Methods and Practices in Psychological Science, researcher Jeffrey Rouder of the University of California, Irvine and colleagues use principles drawn from high-risk fields to propose best practices for minimizing mundane mistakes in psychology labs.
In the article, the authors emphasize that although mistakes may seem inconsequential compared with other methodological issues, researcher error should be taken seriously. First, mistakes are important because they are common. One analysis of the psychology literature found that about half of the articles published over a 30-year period had at least one inaccurately stated statistical test result, meaning that the test statistic and degrees of freedom did not match the p-value. Second, simple errors may produce a bias in the literature, as researchers may tend to check their work for mistakes more rigorously when the results of a study do not support the original hypothesis than when they are in the anticipated direction.
The authors suggest that best practices for curtailing mistakes can be borrowed from high-reliability organizations in high-risk fields such as aviation and medicine. Errors made in these lines of work can have drastic consequences, and researchers have dedicated considerable time and attention to preventing such errors. Although psychology labs may not have the same high stakes as a setting like a nuclear power plant, the principles – and applied practices – that originate in high-reliability organizations are still informative.
Using high-reliability organizations as a guide, the authors outline principles for reducing mistakes, along with practices that can help researchers apply the principles in a lab setting.
One key principle is a preoccupation with failure. In a high-risk field, organizations try to identify future failures and potential mistakes and analyze how to avoid them. Labs can adopt this convention by treating near misses as seriously as full-blown mistakes and taking a proactive approach to anticipate failures.
One practice that can help authors apply this principle in analyzing and displaying their data is using a code-based system. Some analysis software is menu-driven, such as Excel, which means that researchers have to make successive choices when running an analysis or creating a graph, including selecting an option in a menu and copying and pasting cells. A menu-based system doesn’t record those actions, meaning that lab members may be unable to recreate the analysis or graph in the future. To improve reliability, research teams can use a software program that either has a code-based system or both menu- and code-driven analyses. In systems like SPSS, code can be saved and shared so that other researchers can replicate all steps of the analysis.
Learn more about principle and practices for minimizing mistakes in the full article.
Reference
Rouder, J. N., Haaf, J. M., & Snyder, H. K. (2019). Minimizing mistakes in psychological science. Advances in Methods and Practices in Psychological Science. https://doi.org/10.1177%2F2515245918801915
APS regularly opens certain online articles for discussion on our website. Effective February 2021, you must be a logged-in APS member to post comments. By posting a comment, you agree to our Community Guidelines and the display of your profile information, including your name and affiliation. Any opinions, findings, conclusions, or recommendations present in article comments are those of the writers and do not necessarily reflect the views of APS or the article’s author. For more information, please see our Community Guidelines.
Please login with your APS account to comment.