Children’s Preference for Learning Could Help Create Curious AI
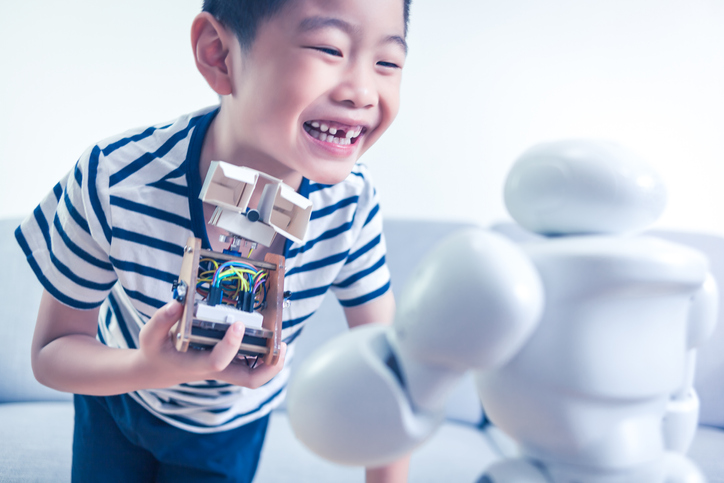
Children are often characterized as behaving randomly, leading them to explore the world in ways that would never occur to adults’ more inflexible minds — but these strategies follow a distinct set of rules, write Eric Schulz (Harvard University) and colleagues in Psychological Science. These characteristics are part of what makes children so valuable to computer scientists looking to create more sophisticated forms of artificial intelligence.
Unlike an algorithm designed to operate in a truly random fashion, children display a preference for exploring highly uncertain features of their environment, the researchers write. This means that while adults may prefer exploiting strategies with a known payoff, children often choose learning at the cost of immediate rewards.
“To fulfill Alan Turing’s dream of creating a childlike artificial intelligence, we need to incorporate generalization and curiosity-driven exploration mechanisms,” explain Schulz and colleagues Charley M. Wu, Azzurra Ruggeri, and Björn Meder (Max Planck Institute for Human Development, Germany).
Schulz and colleagues explored the differences in how adults and children respond to this exploration-exploitation dilemma through a study of 55 children ages 7 to 8, 55 children ages 9 to 11, and 50 adults ages 18 to 55.
Participants, recruited at the Berlin Natural History Museum in Germany, were presented with a series of 10 Minesweeper-like tasks, in addition to several tutorial rounds, in which they were allotted 25 clicks to locate reward tiles. Tiles with similar rewards were clustered together, with reclicked tiles awarding an average of all previous clicks. The participants’ final score was then calculated based on the ratio of their average score to the highest possible score.
Overall, adults scored higher than children in either age group, with older children slightly outperforming their younger peers. The primary difference in their search strategies, the researchers found, was that adults tended to sample more locally from the clustered rewards, while children selected more unique options throughout the task.
“Adults learned faster, whereas children explored more extensively,” Schulz and colleagues explain.
Using computational modeling, the researchers were able to determine that these differences arise in part from adults’ tendency to generalize about stimuli more than children. Adults’ more complex cognitive processing abilities allow them to apply information gleaned from previous experiences to their future actions more effectively, the researchers explain. Over time, children’s ability to apply existing concepts to new tasks, and to update those concepts, continues to develop, which enhances their ability to detect these kinds of regularities.
“Our results shed new light on the developmental trajectories in generalization and exploration, casting children not as merely prone to more random sampling behavior but as directed explorers who are hungry for information in their environment,” Schulz and colleagues conclude.
Reference
Schulz, E., Wu, C. M., Ruggeri, A., & Meder, B. (2019). Searching for rewards like a child means less generalization and more directed exploration. Psychological Science, 30(11), 1561–1572. doi: 10.1177/0956797619863663
APS regularly opens certain online articles for discussion on our website. Effective February 2021, you must be a logged-in APS member to post comments. By posting a comment, you agree to our Community Guidelines and the display of your profile information, including your name and affiliation. Any opinions, findings, conclusions, or recommendations present in article comments are those of the writers and do not necessarily reflect the views of APS or the article’s author. For more information, please see our Community Guidelines.
Please login with your APS account to comment.