Presidential Column
Three Mediation Stories, Three Analytic Strategies
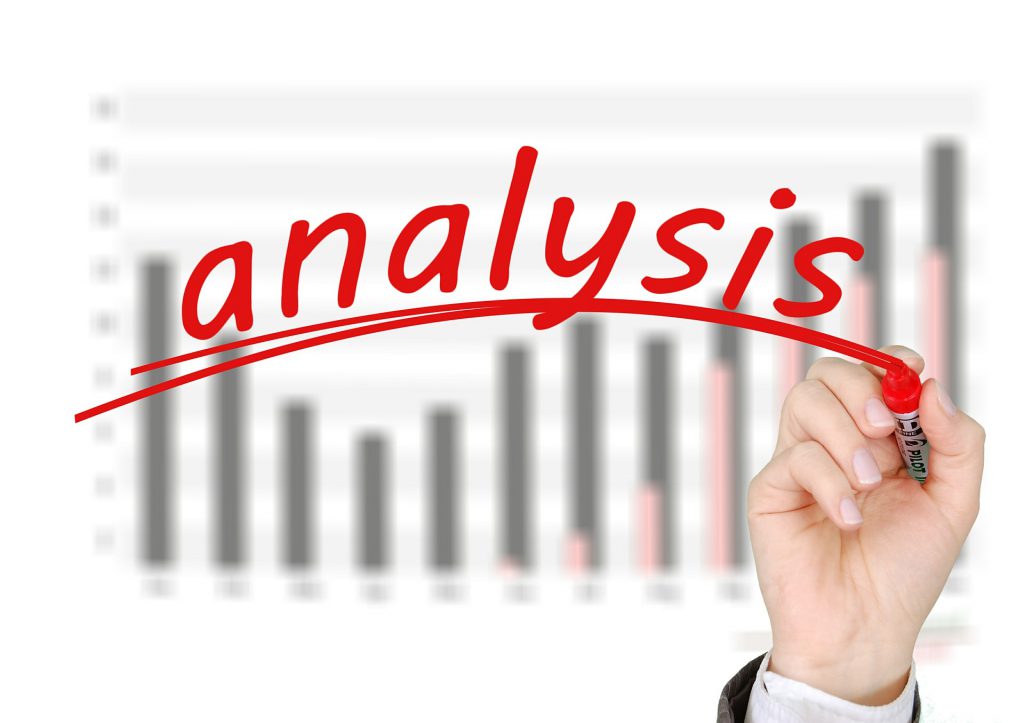
APS is launching a new journal, Advances in Methodologies and Practices in Psychological Science. One goal of the journal is to make methodological advances in psychology and neighboring disciplines accessible to researchers across all areas of psychological science so that each area does not have to reinvent the wheel. In that spirit, I asked Steve Raudenbush and Guanglei Hong, two of my collaborators and colleagues at the University of Chicago, to take a look at three scenarios that psychological researchers typically treat using path analysis. Although superficially similar, the scenarios differ at the core. Acknowledging these differences has led to new statistical techniques — techniques that we hope will find their way into all areas of psychological science.
-APS President Susan Goldin-Meadow
We use this column to highlight three cases of mediation, which seem, on the surface, to call for the same kind of statistical treatment: path analysis (see Figure on p. 6), which was invented by Wright (1934), elaborated by Duncan (1966), and developed for psychology by Baron and Kenny (1986). New thinking about causation suggests that these three problems are not the same. Explaining why points to new methods for tackling problems of mediation.
Case 1: Does bedtime reading (M) mediate the relation between parent education (Z) and child reading achievement (Y)? Case 1 is comparatively simple because there is only one causal variable — bedtime reading. Parent education is not a causal variable.
To understand what is and what is not a causal variable, we have to think about potential outcomes that could counterfactually be achieved under alternative courses of action. Our data provide no information on how children of parents with low education would fare if they had counterfactually been assigned to highly educated parents unless we study adoptions or a special intervention that raises parents’ education levels. If neither adoption nor an intervention that educates parents is a focus of interest, the relationships involving parent education are questions of association, not causation.
Bedtime reading is a causal variable — if we are willing to assert that parents may or may not read to their children at bedtime. In this case, each child possesses two counterfactual achievement outcomes: the outcome we would see if the child were read to, and the outcome we would see if the child were not read to.
The hurdle in answering Question 1, then, is nailing down the causal effect of bedtime reading. Without random assignment of children to the bedtime reading condition, the challenge arises from confounding — even among parents at the same education level, those who read to their children and those who do not may differ in other ways that predict reading achievement. Therefore, measuring and controlling the relevant confounding variables becomes essential. But if we can nail down the causal effect of bedtime reading, we can then simply estimate how much of the association between parent education and reading is explained by access to the causal agent, bedtime reading (Baron & Kenny, 1986).
But there is a caveat. Children of highly educated parents may benefit more from bedtime reading than do children of less educated parents, perhaps because highly educated parents engage children in discussions that facilitate reading comprehension. Here, parent education moderates the association between the causal variable and the outcome. The economist Oaxaca (1973) provides a model for how to deal with this issue in his study of gender gaps in earnings.
Case 2: Does decreasing exposure to violence (M) mediate the relation between moving to an affluent neighborhood (Z) and maternal depression (Y)? Case 2 differs from Case 1 because it involves two causal variables. Z is causal because one might or might not move to a new neighborhood. M is causal because one might or might not be exposed to high levels of violence. Exposure to violence at least partially mediates the impact that moving to an affluent neighborhood has on depression if moving reduces the risk of exposure and if exposure affects depression. In path analysis, by regressing M on Z and regressing Y on M and Z, we obtain an estimate of ab as the indirect effect and c as the direct effect (see Figure).
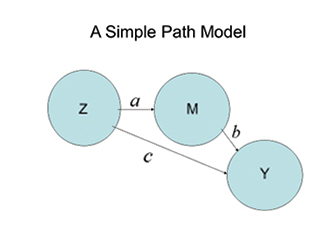
But this standard approach does not work well for Case 2 if the impact of exposure to violence on depression depends on whether one moves. In addition, we also have to worry about confounding, not only because those who move might be different from those who stay at baseline, but also because those who are exposed to violence may differ from those who are not exposed (Bullock, Green, & Ha, 2010; Holland, 1988).
Imai, Tingley, and Yamamoto (2013) discuss how to design an experiment for Case 2, and two recent books summarize new statistical methods for cases where random assignment of the mediator is impossible. These new methods allow treatment-by-mediator interaction and emphasize removal of confounding. VanderWeele (2015) focuses on regression-based approaches, and Hong (2015) focuses on nonparametric models employing propensity score-based weighting (see also foundational work by Pearl, 2001, and other new analytic strategies, e.g., Imai, Keele, & Tingley, 2010).
Case 3: Does cognitive skill (M) mediate the relation between college attendance (Z) and earnings (Y)? Case 3 involves one causal variable — college attendance — and two outcomes — one proximal (cognitive skill) and the other distal (earnings). College attendance is causal because a person may or may not go to college. Cognitive skill is not causal because one cannot be assigned or choose to have high skill; it is instead a “surrogate marker” for earnings. If prior research indicates that cognitive skill (which can be measured early) is a good predictor of later earnings, we may infer the impact of college attendance on earnings even before participants are old enough to work.
Case 3 poses a tough inferential problem even though it entails only one causal variable. To say that cognitive skill accounts for the impact of college attendance on earnings is to dismiss the possibility that college attendance can have a large effect on earnings even for people whose cognitive skill is unaffected by college attendance.
This idea cannot be tested via regression but can be tested through principal stratification (Frangakis & Rubin, 2002). We might classify participants into three principal strata — one for those whose cognitive skill would increase a great deal if they attend college, a second for those whose skill would not increase much even as a result of attending college, and a third for those whose skill would increase even without attending college. If we find that college attendance strongly increases the earnings of persons in the second and third strata, that evidence will falsify the claim that college attendance improves earnings solely by increasing cognitive skill.
But unless we have a crystal ball, we can’t know a priori which stratum to put a person in. Nevertheless, by collecting pretreatment variables that predict college attendance and cognitive skill, we may be able to identify causal effects within each stratum (see Gallop et al., 2009; Page, 2012; commentaries in the same issue; Rubin, 2004). This approach does not constitute a full mediation analysis, but it does put some strong claims of mediation to an important test.
References
Baron, R. M., & Kenny, D. A. (1986). The moderator-mediator distinction in social psychology research: Conceptual, strategic, and statistical considerations. Journal of Personality and Social Psychology, 51, 1173–1182.
Bullock, J. G., Green, D. P., & Ha, S. E. (2010). Yes, but what’s the mechanism? (Don’t expect an easy answer). Journal of Personality and Social Psychology, 98, 550–558.
Duncan, O. D. (1966). Path analysis: Sociological examples. American Journal of Sociology, 72, 1–16.
Frangakis, C. E., & Rubin, D. B. (2002). Principal stratification in causal inference. Biometrics, 58, 21–29.
Gallop, R., Small, D. S., Lin, J. Y., Elliott, M. R., Joffe, M., & Ten Have, T. R. (2009). Mediation analysis with principal stratification. Statistics in Medicine, 28, 1108–1130.
Holland, P. (1988). Causal inference, path analysis, and recursive structural equations models. Sociological Methodology, 18, 449–484.
Hong, G. (2015). Causality in a social world: Moderation, mediation and spill-over. Sussex, United Kingdom: Wiley.
Imai, K., Keele, L., & Tingley, D. (2010). A general approach to causal mediation analysis. Psychological Methods, 15, 309–334.
Imai, K., Tingley, D., & Yamamoto, T. (2013). Experimental designs for identifying causal mechanisms. Journal of the Royal Statistical Society: Series A (Statistics in Society), 176, 5–51.
Oaxaca, R. (1973). Male-female wage differentials in urban labor markets. International Economic Review, 14, 693–709.
Page, L. C. (2012). Principal stratification as a framework for investigating mediational processes. Journal of Research on Educational Effectiveness, 5, 215–244.
Pearl, J. (2001). Direct and indirect effects. Proceedings of the American Statistical Association Joint Statistical Meetings, 1572–1581.
Rubin, D. B. (2004). Direct and indirect causal effects via potential outcomes. Scandinavian Journal of Statistics, 31, 161–170.
VanderWeele, T. J. (2015). Explanation in causal inference: Methods for mediation and interaction. Oxford, United Kingdom: Oxford University Press.
Wright, S. (1934). The method of path coefficients. Annals of Mathematical Statistics, 5, 161–215.