How to Maintain Data Quality When You Can’t See Your Participants
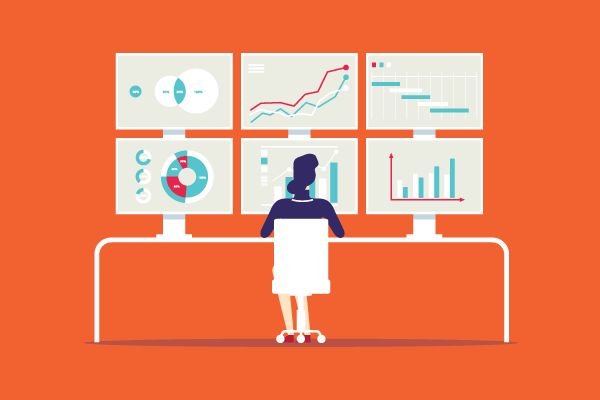
Collecting your first dataset using online recruitment can be fabulous and disconcerting in equal measure. After weeks (or months, or years) of careful experimental design and stimulus prep you click the “begin data collection” button and then head off for lunch. Or, if you are like me, you sit obsessively watching the ‘number of complete datasets’ counter click inexorably upwards. In contrast to the many hours of waiting for participants that are usually associated with lab-based experiments, this new form of remote experimentation can seem magically wonderful.
And yet it also feels, at least to me, that something is just not quite right. As an experimental psychologist, behavioural data is the cornerstone of our research and it can feel deeply disconcerting for our data to arrive onto our computer without us being able to directly observe its creation: We have no virtual spyhole on the door of the testing cubicle with which to monitor our participants’ performance.
Broadly speaking, the many advantages of online data collection fall into two categories. First, it reduces the time that researchers must spend recruiting and testing participants: Crowdsourcing platforms such as Prolific Academic (www.prolific.ac) and Mechanical Turk (www.mturk.com) allow large numbers of participants to be recruited at the click of a button. Second, and in my view more importantly, this approach allows us to move away from testing the relatively restricted populations of university undergraduates who are most easily recruited for lab-based experiments. It is now much easier to recruit more demographically balanced samples, and to target specific populations that might be difficult to find or recruit via more conventional means.
But these clear advantages come at a price. Many researchers are deeply concerned about the methodological consequences of remote testing where we cannot directly observe our participants. In conventional experiments, the researcher typically meets each participant and has a face-to-face (albeit brief) chat before the experiment begins. This allows us to verify some of their basic demographic information. We can confirm that they have not already participated in our experiment and can speak our chosen language fluently. The experiment then typically takes place in a quiet room where all participants complete the experiment free from distraction using the same carefully selected equipment.
In contrast, when we run our experiments online we necessarily give up much of this experimental control and must accept a much higher degree of uncertainty about (i) who our participants are and (ii) the conditions under which the experiment is being conducted.
New Possibilities
Despite this apparent lack of control, my experiences with online data collection have been overwhelmingly positive. This approach has allowed us to run experiments that could not possibly have been implemented within the lab, either because they required an unfeasibly large number of participants, or because we wanted to recruit very specific participants who did not all live in central London (see https://jennirodd.com/publications/). And despite the magical method by which our data arrived, our data in most cases have turned out to be highly informative.
Additionally, over the last 5 years we have developed methods that have greatly improved our data quality. There are several important steps that experimenters can take to maximise their data quality. First and foremost, you should take great care when selecting the source of participants — when using a crowdsourcing platform, it is important to check their processes for recruiting and screening participants. And if recruiting via more informal social media routes, think very carefully about how these participants might differ from those recruited by more conventional approaches.
Second, make sure you reward your participants appropriately. If they feel you do not really value their time, then they will, in turn, not value your experiment and your data quality will likely suffer.
While these two general pieces of advice are a good starting place, I suggest that to really be able to trust the data quality for any online experiment, we must explicitly adapt our experimental paradigms to fit the online world.
Importantly, I’ve learned that there are no magic bullets that can be applied across the board to safeguard every online experiment that we might want to run. Each experiment is different and we need to tailor the safeguards that we include according to our specific experimental method and the particular hypotheses being tested. I therefore suggest that researchers step through the following five-stage process prior to collecting data in any specific online experiment.
1. Specify your data quality concerns
The first, and perhaps most critical step, is to explicitly specify any concerns that you might have about how moving to online data collection could potentially ruin your experiment. What could possibly go wrong? In general, these concerns tend to fit into three categories.
- Where are participants doing the experiment?
You will almost certainly worry that participants may be working in a noisy, distracting environment in which they may not properly attend to your (dull?) experiment. They may, for example, be “multiscreening” to check their social media. Also participants may be using low-quality hardware (slow internet connections, small screen, poor-quality headphones, etc.).
- Are participants who they say they are?
You may be concerned that participants might lie about their age, language proficiency, background, or some other important demographic factor. Think carefully about the likelihood of these problems, paying particular attention to any reward systems that might exacerbate them. If you are paying participants relatively well, for example, then people who are ineligible to take part may lie to gain access. Alternatively, if your experiment is a super-fun online game but only open to people 18-years-old and above, then children may lie about their age to gain access.
- Are they cheating on the task?
Finally, you may be concerned about participants’ behaviour during the experiment itself. They may, for example, look up the answers to your questions on Google — something they couldn’t do if you were watching them in the lab. Memory experiments can be particularly problematic: It can be difficult to ensure that participants are not writing down or screen-grabbing the information they are supposed to remember. Again, think carefully about the incentives that might drive participants to cheat — is their payment or their ability to stay on the participant database in some way contingent on their performance
2. Specify the worst case scenario
For all the above concerns, it is critical to think through the worst case scenario for your particular experiment. While some of the issues you have identified in Stage 1 might simply add a bit of noise to your data — and can be counteracted by collecting sufficient data or by careful analysis — other issues could potentially be catastrophic. No journal is going to publish your working-memory experiment if it seems likely that participants were writing down the correct answers. And no journal will publish your experiment showing that monolinguals and bilinguals perform equally on some critical test of language processing unless you can securely demonstrate that participants were correctly assigned to these two groups. In some cases, this might be the point where you abandon your plan to collect data online and return to your lab-based protocol. But in my experience the vast majority of issues are fixable.
3. Add new within-experiment safeguards
At this point, you should make every effort to tweak your existing experimental design to improve your data quality. To be honest, there is often not much that can be done. But imposing sensible time limits for the different stages of your task can help increase the likelihood that participants (i) stay on task and (ii) refrain from cheating. It is now also relatively straightforward on most experimental platforms to screen out participants on the basis of their hardware/software — this can be particularly important for auditory experiments in which you want to ensure that they are using headphones as instructed.
4. Design experiment-specific exclusion criteria
The next, critical step is accepting that you will inevitably collect some data that will be unusable — you simply cannot ensure that all participants will behave as instructed. It is therefore necessary to devise a set of experiment-specific criteria for excluding participants’ datasets from your analyses. Each of these should relate directly to a specific concern that was set out in stage 1 — it is vital to keep in mind exactly why you are including each criterion.
- Set performance criteria for existing tasks
In many cases, you can set these criteria using the data that you already plan to collect. For example, if your priority is to ensure that participants are adequately attending to your key task, then it is often sufficient to collect accurate reaction times and exclude participants with long or variable responses. You may also wish to ensure that adequate time was spent reading the instructions. Other more sophisticated methods that check for expected patterns of variance or entropy in the data are also feasible. For new tasks, pilot data can allow you to characterise the typical range of participant performance — this is often best collected in the lab where you can observe participants and obtain more detailed feedback.
- Set criteria for additional tasks/measures
In some cases, you will need to collect additional data to know who you should reasonably include in your analysis. For example, if you want to verify participants’ proficiency in different languages then you may need to add a short, timed vocabulary test and specify the minimum requirements needed for a participant’s data set to be included. Sometimes, it can be worth testing or questioning a key demographic more than once and excluding participants that give inconsistent responses.
5. Pre-register your exclusion criteria
Finally, I believe it is really important to preregister these (sometimes complex) exclusion criteria prior to data collection. In some cases, such as studies that involve lengthy and boring experiments, you may need to exclude significant numbers of participants and if you haven’t preregistered these criteria then the scientific community has no way to confirm that you didn’t “cherry-pick” the participants that contribute to a nice statistical outcome.
But of course, even the best preregistration documents cannot possibly foresee all the possible ways in which participants can mess up your experiment. We sometimes end up with data from participants who meet all our criteria but who most reasonable researchers would agree should be excluded from the analysis (e.g., a participant who performs reasonably well on the task but then tells you that he was drunk and had not slept for 3 days). In such cases, it is reasonable to deviate from your preregistration document as long as you are completely transparent about your actions and reasoning.
Moving Back to the Lab
It is important to note that nothing in the process is specific to online experiments. Indeed, this approach could also help us improve the quality of our lab-based experiments. Although some of the issues (e.g., quality of hardware) don’t arise in this context, the vast majority can — especially when participants are left unsupervised. Can we really be certain that our lab participants are not looking at pictures of cute cats on their phone at the same time they’re completing our tasks?
The move to online experiments has improved the quality of my lab-based experiments, as I now consider in far greater detail than before the process by which I reassure myself, and my peers, about the quality of the data that I have collected.
Watch a video of Rodd’s recent presentation on this topic here.
References and Further Reading
Clifford, S., & Jerit, J. (2014). Is there a cost to convenience? An experimental comparison of data quality in laboratory and online studies. Journal of Experimental Political Science, 1(02), 120–131. doi.org/10.1017/xps.2014.5
Crump, M. J. C., McDonnell, J. V., & Gureckis, T. M. (2013). Evaluating Amazon’s Mechanical Turk as a tool for experimental behavioral research. PLoS ONE, 8(3), e57410. doi.org/10.1371/journal.pone.0057410
Munafò, M. R., Nosek, B. A., Bishop, D. V. M., Button, K. S., Chambers, C. D., Percie du Sert, N., … Ioannidis, J. P. A. (2017). A manifesto for reproducible science. Nature Human Behaviour, 1(1), 0021. doi.org/10.1038/s41562-016-0021
Reimers, S., & Stewart, N. (2015). Presentation and response timing accuracy in Adobe Flash and HTML5/JavaScript Web experiments. Behavior Research Methods, 47(2). doi.org/10.3758/s13428-014-0471-1
Rodd, J. M., Cai, Z. G., Betts, H. N., Hanby, B., Hutchinson, C., & Adler, A. (2016). The impact of recent and long-term experience on access to word meanings: Evidence from large-scale internet-based experiments. Journal of Memory and Language, 87, 16–37. https://doi.org/10.1016/j.jml.2015.10.006
Stewart, N., Chandler, J., & Paolacci, G. (2017). Crowdsourcing samples in cognitive science. Trends in Cognitive Sciences, 21(10), 736–748. doi.org/10.1016/J.TICS.2017.06.007
Woods, A. T., Velasco, C., Levitan, C. A., Wan, X., & Spence, C. (2015). Conducting perception research over the internet: a tutorial review. PeerJ, 3, e1058. doi.org/10.7717/peerj.1058
Woods, K. J. P., Siegel, M. H., Traer, J., & McDermott, J. H. (2017). Headphone screening to facilitate web-based auditory experiments. Attention, Perception, and Psychophysics. doi.org/10.3758/s13414-017-1361-2
Comments
For those interested more in running online studies, we have published a preprint on biorxiv about Gorilla.sc an experiment builder, we also give an overview of other tools for building an online research (https://www.biorxiv.org/content/10.1101/438242v4)
While pre-registering exclusionary criteria could act as a safe guard to prevent cherry picking, I would disagree that it should be registered prior to data collection for several reasons.
1. It is not methodologically practical. If you have the time and opportunity to pilot your survey, that is fantastic! However, if you do not, you may find weird nuances with the data which you did not anticipate. Maybe you have accuracy as a primary DV but you selected items that were too hard. If you established a min. performance threshold, you’d have to exclude all your data.
2. Often, even if you do pilot, you may find participants have ways to cheat a system, or still provide irregular response patterns that do not make a lot of sense (such as suicidal behavior but no suicidal thoughts or depression). Whether or not you would include these responses depends on a lot of things, ranging from whether your hypotheses are geared toward a more prototypical form of depression.
3. There are better (more systematic) ways which do not include a need for establishing your own cut-offs. For instance, there are a lot of techniques such as using IRT to detect aberrant response patterns (see recent work by RR Meijer), or conducting median splits and mean trimming, which may or may not make sense depending on the type of items you select and the distributions you’re seeing across your data. To some degree, you could specify general techniques you may employ, but it is even difficult to do that prior to data collection.
4. It is not logistically practical. It would add a lot of time for people submitting grants to have to flush out and define their exclusion criteria ahead of time. I am not saying this means we should not do it, just that this would be a huge barrier to overcome if you wanted people to adopt that suggestion.
5. It is not always practical from a staffing standpoint. Having to define validation rules prior to data collection is something people should do, as this helps ensure their survey is well designed (sort of like making sure you have manipulation checks in your experiment). However, often labs hire external staff to manage data and sometimes even conduct analyses. You could argue they could hire the data managers prior to data collection to establish the systems and data libraries, but this often means having to pull someone off other work to do cursory set-up and help define rules for a grant which may not even get funded.
In an ideal world your suggestion is great, but I just feel in practice it cannot work with many current systems.
This article is super helpful — I’ve met so many researchers who avoid running studies online because of data quality concerns.
We recently updated our preprint ‘Gorilla in our midst: An online behavioural experiment builder'( doi.org/10.1101/438242 ) with a comparison of tools to run studies and discuss controlling for data quality.
APS regularly opens certain online articles for discussion on our website. Effective February 2021, you must be a logged-in APS member to post comments. By posting a comment, you agree to our Community Guidelines and the display of your profile information, including your name and affiliation. Any opinions, findings, conclusions, or recommendations present in article comments are those of the writers and do not necessarily reflect the views of APS or the article’s author. For more information, please see our Community Guidelines.
Please login with your APS account to comment.