Featured
Up-and-Coming Voices: Artificial Intelligence in Psychological Science
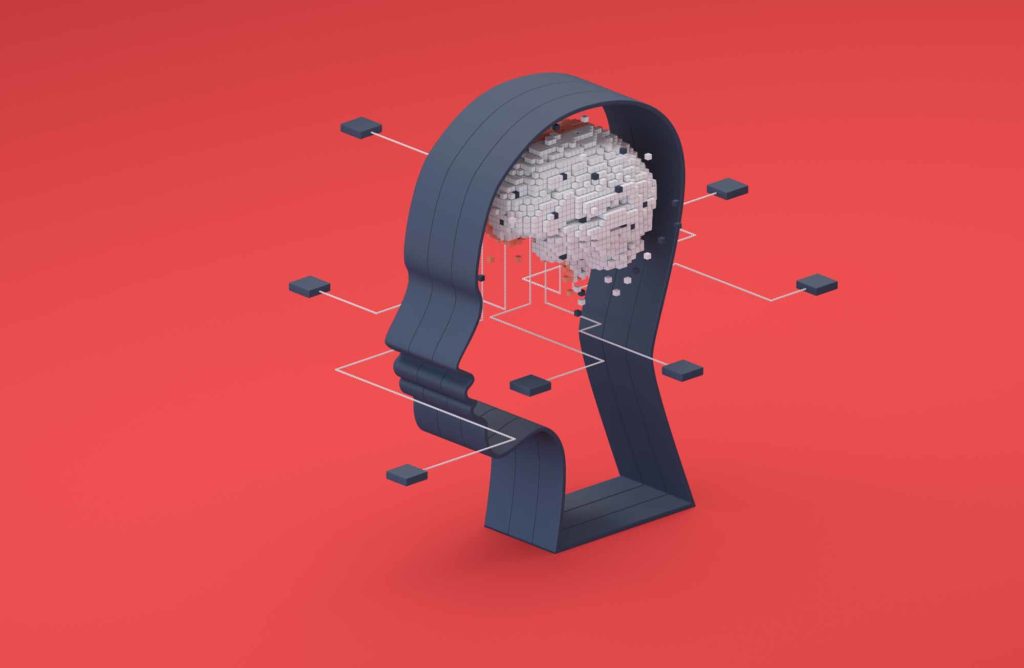
Role of Emotional Tone and Gender of Computer-Generated Voices in Multimedia Lessons • Qualitative and Quantitative Assessments of Topic Gists Extracted Using Machine Learning from Social Media Messages about COVID-19 • Predicting Developmental Delay in Very Pre-Term Infants Using Machine Learning
At the 2022 APS Annual Convention in Chicago last May, students and early-career researchers had an opportunity to network and present their research to the scientific community through poster sessions. For this issue, we’ve asked three student researchers to share their research, their findings, and their next steps into work relating to artificial intelligence (AI) and psychological science.
Role of Emotional Tone and Gender of Computer-Generated Voices in Multimedia Lessons
Fangzheng Zhao and Richard E. Mayer (University of California, Santa Barbara)
What did the research reveal that you didn’t already know?
The cognitive-affective model of learning with media claims that learners can perceive and react to the emotional stance displayed by the instructors: That is, students’ felt emotions, social partnership feelings with the instructor, and the learning outcomes can be influenced by instructors’ emotional stance. This research revealed that besides real human voices, synthesized voices with different gender (female, male) and various emotional tones (e.g., happy, sad) could also affect students’ perceived emotions, felt emotions, and social connections with the instructors in video lessons. In addition, different from the matching hypothesis, female and male students’ learning experiences and outcomes were not moderated by the gender of the synthesized voices.
How might your findings contribute to the broader research on artificial intelligence and psychological science?
As a contribution to future work on artificial intelligence and psychological science, this research showed the prospects of using AI-synthesized voices as instructors’ voices in video lessons as an economical, convenient, and—most importantly—effective option as a replacement for real human instructors. This study also revealed the need for future research on how to design instruction with disembodied synthesized affective voices that can convey emotion in ways that promote learning processes and outcomes.
What are your next steps regarding this research?
We would like to try more advanced machine voice generators and conduct pre-tests to control the emotional arousal level of each type of generated voice to see whether those changes can provide a better experience and improved learning outcomes for students. In addition, we are also interested in investigating whether the results might change if we add the embodied onscreen instructor (AI-generated virtual agents vs. real human instructors), rather than just voices (AI generated machine voices vs. real human voices), in the video lessons.
Qualitative and Quantitative Assessments of Topic Gists Extracted Using Machine Learning from Social Media Messages about COVID-19
Megan A. Birmingham, Valerie F. Reyna, Demetrius Bryson, and Sarah M. Edelson (Cornell University), and David A. Broniatowski (George Washington University)
What did the research reveal that you didn’t already know?
We learned that millions of social media messages about important health topics related to COVID-19 could be summarized using machine learning models and that human judges could evaluate these models. Fuzzy-trace theory allowed us to meaningfully interpret all of this. We identified two “gist” themes that we examined in depth: (1) social distancing/lockdowns and (2) relationships between COVID-19 and the flu. Crucially, as anticipated by fuzzy-trace theory, the gists human judges took away from machine-generated topics were informed by personal experience with the pandemic and prior knowledge. Overall, we learned how people were thinking about and understanding social media messages, specifically at a critical stage of COVID-19—right when it was declared a pandemic.
How might your findings contribute to the broader research on artificial intelligence and psychological science?
We used machine learning algorithms that extracted the gist of millions of social media messages. Our project focused on two topics, but the research overall represents the first step in systematically evaluating these AI models. The two topics we focused on were related to risk reduction for COVID-19 and other viral outbreaks. We can use the knowledge gained here to aid in the larger effort of increasing automated gist elicitation. This would help public health communicators to quickly understand which gists should be communicated during outbreaks, how to communicate them, and which communities they should be communicated to.
Related content: Science in Service: Leveraging Psychological Science to Put the “Public” in Public Health
What are your next steps regarding this research?
Next steps for this project include analyzing how gist messages evolve over time as perceptions of risks and benefits change. Fuzzy-trace theory will be helping us with that goal because it generates predictions about likely gist topics and about how people communicate about risks (Reyna, 2021, in Proceedings of the National Academy of Sciences, is open access and has details: https://www.pnas.org/doi/10.1073/pnas.1912441117). We hope to use this research to produce messages, with the help of AI systems, that promote risk-reduction behaviors and to improve public health communication about how to express the risks and benefits of behaviors. This research has the potential to empower individuals to make choices that not only mitigate the spread of COVID-19 but also address other public health emergencies in the future.
Predicting Developmental Delay in Very Pre-Term Infants Using Machine Learning
Gozde Merve Demirci (CUNY Graduate Center) and Chia-Ling Tsai (CUNY Queens College), Michael J. Flory (NYS Institute for Basic Research), Ha T. Phan, Anne Gordon, and Phyllis Kittler (NYS Institute for Basic Research in Developmental Disabilities; Richmond University Medical Center), and Santosh Parab (Richmond University Medical Center)
What did the research reveal that you didn’t already know?
The goal of our research was to use machine learning (ML) for earlier detection of neurodevelopmental delays in preterm infants. Our research revealed that even though at-birth clinical variables contribute to model prediction, additional longitudinal features were required to increase model performance. Instead of waiting two years to identify a neurodevelopmental delay in a child, our ML modeling helped to make a prediction earlier by combining at-birth and longitudinal variables. With high specificity, ML managed to predict delays 6 months in advance. Additionally, unlike similar studies, we predicted both cognitive/language and fine/gross motor delays with the same set of ML models. This revealed that the at-birth predictors for both domains closely coincided. Taken together, our findings indicate that ML can help earlier prediction of neurodevelopmental delays at month 25, with at-birth and longitudinal variables.
How might your findings contribute to the broader research on artificial intelligence and psychological science?
Our findings show the challenges that arise from longitudinal medical/developmental data, such as missing values and imbalanced target values, can be addressed using ML. Within ML applications, helpful synthetic data can be created to resolve these challenges and maximize the usefulness of the data so painstakingly collected. Compared to statistical analysis, ML can help generalize results and apply model architecture to a broader range of clinical problems. We believe that applying AI techniques to additional at-birth variables, such as raw cranial ultrasound images, will enhance the accuracy of model predictions requiring fewer long-term longitudinal score variables.
What are your next steps regarding this research?
Our future direction is to understand the essential at-birth clinical variables with the help of using explainable AI techniques and to study each feature’s effect on the target prediction. Also, in our study population, there are eight different longitudinal test scores, obtained every 3 months. We plan to create eight scenarios with these subsequent longitudinal scores. This helps us to analyze if we can use earlier months than month 16 for better prediction of neurodevelopmental delays. We believe that ML implementations have so far been underutilized in psychology, but they do have great potential for the field. Using the power of ML, there is the possibility of better diagnosis and prognosis in clinical studies, and the possibility of better prediction of developmental outcomes.
Feedback on this article? Email apsobserver@psychologicalscience.org or login to comment. Interested in writing for us? Read our contributor guidelines.
APS regularly opens certain online articles for discussion on our website. Effective February 2021, you must be a logged-in APS member to post comments. By posting a comment, you agree to our Community Guidelines and the display of your profile information, including your name and affiliation. Any opinions, findings, conclusions, or recommendations present in article comments are those of the writers and do not necessarily reflect the views of APS or the article’s author. For more information, please see our Community Guidelines.
Please login with your APS account to comment.