New Content from Advances in Methods and Practices in Psychological Science
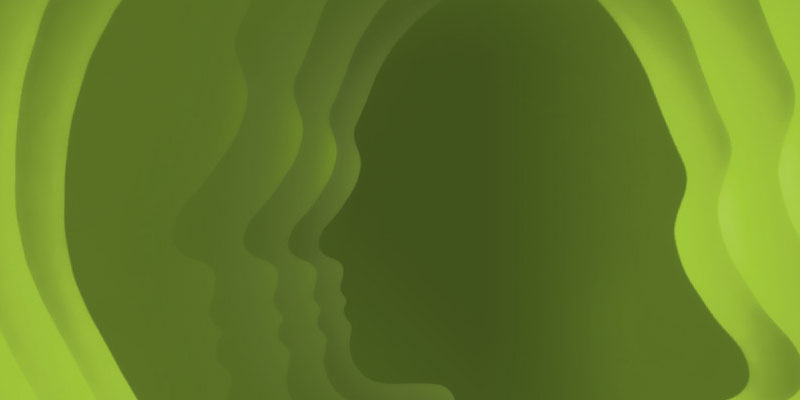
Special Section: Using Simulation to Convey Statistical Concepts
In this special section of Advances in Methods and Practices in Psychological Science, seven tutorials introduce the use of simulations in developing statistical intuitions. Simulations can help students and researchers better grasp statistical principles and the consequences of analysis decisions. This collection can be useful to researchers interested in gaining a better understanding of statistical principles, instructors of undergraduate and graduate statistics classes, and students in those classes.
Selection of the Number of Participants in Intensive Longitudinal Studies: A User-Friendly Shiny App and Tutorial for Performing Power Analysis in Multilevel Regression Models That Account for Temporal Dependencies
Ginette Lafit et al.
This step-by-step tutorial shows how to perform simulation-based power analyses (and select the appropriate number of participants) for models widely used in intensive longitudinal research.
Simulation Studies as a Tool to Understand Bayes Factors
Don van Ravenzwaaij and Alexander Etz
This tutorial provides an accessible way to use a simulation script to approximate the calculation of Bayes factors and to learn about the Jeffreys-Zellner-Siow (JZS) Bayes factor, a popular implementation of this factor used in the freeware program JASP and the BayesFactor package for R.
Understanding Mixed-Effects Models Through Data Simulation
Lisa M. DeBruine and Dale J. Barr
This tutorial can enhance researchers’ understanding of mixed-effects models. It explains how to simulate data with random effects of both subjects and stimuli as well as how to analyze the data using linear mixed-effects regression (with the lme4 package for R).
Simulation-Based Power Analysis for Factorial Analysis of Variance Designs
Daniël Lakens and Aaron R. Caldwell
This tutorial shows researchers how to use the R package Superpower and online Shiny apps that Lakens and Caldwell created to perform simulation-based power analysis for complex analysis of variance (ANOVA) designs of up to three within- or between-participants factors (including power for main effects, interactions, and individual comparisons).
Making Sense of Model Generalizability: A Tutorial on Cross-Validation in R and Shiny
Q. Chelsea Song, Chen Tang, and Serena Wee
This tutorial helps researchers use cross-validation to evaluate model generalizability (i.e., how well the findings from a sample apply to other samples). Song and colleagues use a Shiny app to simulate and visualize how model generalizability is influenced by model complexity, effect size, and sample size.
Power Analysis for Parameter Estimation in Structural Equation Modeling: A Discussion and Tutorial
Y. Andre Wang and Mijke Rhemtulla
This tutorial illustrates how to use a Shiny app, pwrSEM, to conduct a power analysis for detecting a target effect (e.g., a regression coefficient between latent variables) in structural equation models.
The Percentile Bootstrap: A Primer With Step-by-Step Instructions in R
Guillaume A. Rousselet, Cyril R. Pernet, and Rand R. Wilcox
This step-by-step tutorial shows how to use R to implement and assess the behavior of the percentile bootstrap—a nonparametric method based on data-driven simulations—for making inferences about central tendency and spread in different experimental designs.
APS regularly opens certain online articles for discussion on our website. Effective February 2021, you must be a logged-in APS member to post comments. By posting a comment, you agree to our Community Guidelines and the display of your profile information, including your name and affiliation. Any opinions, findings, conclusions, or recommendations present in article comments are those of the writers and do not necessarily reflect the views of APS or the article’s author. For more information, please see our Community Guidelines.
Please login with your APS account to comment.