New Content From Advances in Methods and Practices in Psychological Science
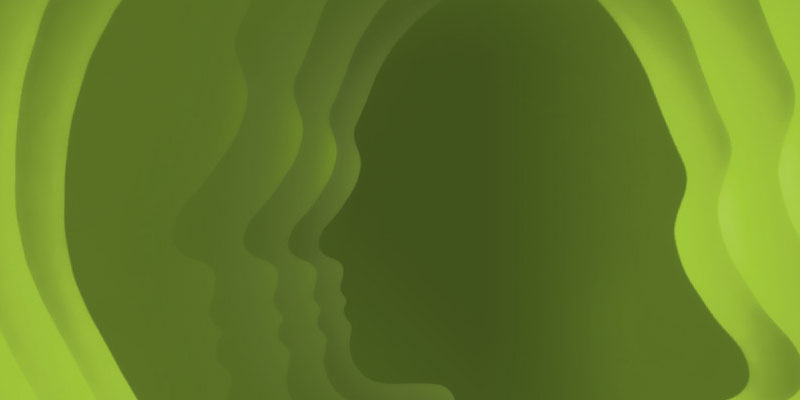
Beyond Random Effects: When Small-Study Findings Are More Heterogeneous
T. D. Stanley, Hristos Doucouliagos, and John P. A. Ioannidis
Evidence from meta-analyses indicates that small-sample studies typically have higher heterogeneity and higher standard errors. This correlated heterogeneity violates the random-effects (RE) model of additive and independent heterogeneity. When small studies have not only inadequate statistical power but also high heterogeneity, their scientific contribution is even more dubious. Simulations show that, in such situations, an alternative weighted average model, the unrestricted weighted least squares (UWLS), outperforms the RE model. Thus, Stanley and colleagues argue that UWLS should replace RE as the conventional meta-analysis summary of psychological research.
Journal N-Pact Factors From 2011 to 2019: Evaluating the Quality of Social/Personality Journals With Respect to Sample Size and Statistical Power
R. Chris Fraley et al.
The N-pact factor, proposed initially by Fraley and Vazire, indexes the median sample size of published studies, providing an indicator of research quality. Fraley and colleagues examined the N-pact factor of social/personality-psychology journals between 2011 and 2019. Results indicated that journals that emphasized personality processes and individual differences had larger N-pact factors than journals that emphasized social-psychological processes. Although the majority of journals in 2011 published studies that were not well powered to detect an effect of ρ = .20, this situation had improved considerably by 2019, suggesting that the field of social/personality psychology has begun to use larger samples.
Comparing Analysis Blinding With Preregistration in the Many-Analysts Religion Project
Alexandra Sarafoglou, Suzanne Hoogeveen, and Eric-Jan Wagenmakers
Sarafoglou and colleagues compared preregistration with analysis blinding—a method in which researchers develop their analysis of a research question on an altered version of the data collected in the original research. In the Many-Analysts Religion Project, 120 teams answered the same research questions with the same data set, either preregistering their analysis or using analysis blinding. Results support the hypothesis that analysis blinding leads to fewer deviations from the analysis plan, and on fewer aspects. Also, both methods required approximately the same amount of time. Thus, analysis blinding does not mean less work, but it does allow researchers to plan more appropriate analyses with fewer deviations.
These Are Not the Effects You Are Looking for: Causality and the Within-/Between-Persons Distinction in Longitudinal Data Analysis
Julia M. Rohrer and Kou Murayama
Rohrer and Murayama aim to show that the relationship between the within- and between-persons distinction and causal inference in longitudinal data analysis is informative but not decisive. They argue that within-persons data are not necessary for causal inference (e.g., between-persons experiments can inform about average causal effects). They also propose that within-persons data are not sufficient for causal inference (e.g., spurious within-persons associations may occur) but can be helpful. Rohrer and Murayama suggest that instead of letting statistical models dictate which questions to ask, researchers should start with well-defined theoretical descriptions of effects to determine study design and data analysis.
Information Provision for Informed Consent Procedures in Psychological Research Under the General Data Protection Regulation: A Practical Guide
Dara Hallinan, Franziska Boehm, Annika Külpmann, and Malte Elson
Informed consent procedures under the European General Data Protection Regulation (GDPR) require providing research participants with specific forms of information. In this tutorial, Hallinan and colleagues offer psychological researchers general guidance about informed consent under the GDPR. The GDPR applies, as a rule, to psychological research conducted on personal data in the European Economic Area—and even, in certain cases, to psychological research conducted on personal data outside this area. Specifically, Hallinan and colleagues suggest that researchers provide information about: types of personal data collected, the controller(s) and recipients of data, the purposes of processing, risks and safeguards, international transfers of data, storage periods, participants’ rights, contractual or statutory requirements, and automated decision-making.
Low Research-Data Availability in Educational-Psychology Journals: No Indication of Effective Research-Data Policies
Markus Huff and Elke C. Bongartz
Huff and Bongartz examined whether educational psychology articles are sharing more data than previously. They coded the availability of research data for 1,242 publications from six educational-psychology journals published in 2018 and 2020 and compared it with data availability in the psychological journal Cognition in the same years. Data availability in educational-psychology journals was overall low both years (3.85% on average compared with 62.74% in Cognition) but increased from 0.32% (2018) to 7.16% (2020). However, there was no relationship between research-data availability and either the journal’s data-transparency level or the existence of an official research-data policy at the corresponding author’s institution.
The Chinese Open Science Network (COSN): Building an Open Science Community From Scratch
Haiyang Jin et al.
In 2016, the Chinese Open Science Network (COSN) was created to reach Chinese-speaking early-career researchers (ECRs) and scholars at large. Since its creation, COSN has grown from a small open science interest group to a recognized network in both the Chinese-speaking research community and the international open science community. As of July 2022, COSN had organized three in-person workshops, 12 tutorials, 48 talks, and 55 journal club sessions and translated 15 open science-related articles and blogs from English to Chinese. The main social media account of COSN has more than 23,000 subscribers, and more than 1,000 researchers/students actively participate in the discussions on open science. Jin and colleagues share their experience building COSN and encourage ECRs in developing countries to start their own open science initiatives and engage in the global open science movement.
A Guide for Calculating Study-Level Statistical Power for Meta-Analyses
Daniel S. Quintana
In this tutorial, Quintana introduces the metameta R package and app, which facilitate the straightforward calculation and visualization of study-level statistical power in meta-analyses for a range of hypothetical effect sizes. The statistical power of a study’s design/statistical test combination for detecting hypothetical effect sizes of interest determines a study’s evidential value, and the credibility of a meta-analysis depends on the evidential value of the studies included. Quintana shows how to reanalyze data using information typically presented in meta-analysis forest plots or tables and how to integrate the metameta package when reporting novel meta-analyses. The researcher also provides a step-by-step companion screencast video tutorial to assist readers using the R package.
Why the Cross-Lagged Panel Model Is Almost Never the Right Choice
Richard E. Lucas
The cross-lagged panel model (CLPM) is a technique for examining reciprocal causal effects using longitudinal data. Critics of the CLPM have noted that, by failing to account for certain person-level associations, estimates of these causal effects can be biased. This critique led to the development of modern alternatives; however, some researchers still defend the CLPM as a better model. Lucas discusses the ways that these defenses of the CLPM fail to acknowledge well-known limitations of the model. The researcher shows in simulated data that the CLPM is likely to either find spurious inexistent cross-lagged effects or underestimate them.
Evaluating Implementation of the Transparency and Openness Promotion Guidelines: Reliability of Instruments to Assess Journal Policies, Procedures, and Practices
Sina Kianersi et al.
The Transparency and Openness Promotion (TOP) Guidelines describe modular standards that journals can adopt to promote open science. Kianersi and colleagues examined the interrater agreement and reliability of three instruments for assessing TOP implementation in journal policies (instructions to authors), procedures (manuscript-submission systems), and practices (journal articles), in 339 journals from the behavioral, social, and health sciences. Interrater agreement (IRA) was high for most standards, but most journals did not implement most TOP standards. No standard had “excellent” interrater reliability (IRR). Three standards had “good,” one had “moderate,” and six had “poor” IRR. Likewise, IRA was high for most questions, and IRR was moderate or worse for 62%, 54%, and 43% of policy, procedure, and practice questions, respectively. Kianersi and colleagues suggest that clarifying distinctions among different levels of implementation for each TOP standard might improve its implementation and assessment.
A Primer on Structural Equation Model Diagrams and Directed Acyclic Graphs: When and How to Use Each in Psychological and Epidemiological Research
Zachary J. Kunicki, Meghan L. Smith, and Eleanor J. Murray
Kunicki and colleagues provide a guide on the distinctions between model diagrams used with structural equation models (SEMs) and causal directed acyclic graphs (DAGs). Both types of diagrams share visual similarities, but SEM diagrams are conceptual and statistical tools in which models are drawn and then tested. By comparison, causal DAGs are exclusively conceptual tools used to help guide researchers in developing analytic strategies and interpreting results. Kunicki and colleagues offer high-level overviews of SEMs and causal DAGs; they then compare and contrast the two methodologies and describe when each would be used. They provide sample analyses, code, and write-ups for both SEM and causal DAG approaches.
Feedback on this article? Email apsobserver@psychologicalscience.org or login to comment. Interested in writing for us? Read our contributor guidelines.
APS regularly opens certain online articles for discussion on our website. Effective February 2021, you must be a logged-in APS member to post comments. By posting a comment, you agree to our Community Guidelines and the display of your profile information, including your name and affiliation. Any opinions, findings, conclusions, or recommendations present in article comments are those of the writers and do not necessarily reflect the views of APS or the article’s author. For more information, please see our Community Guidelines.
Please login with your APS account to comment.