New Content from Advances in Methods and Practices in Psychological Science
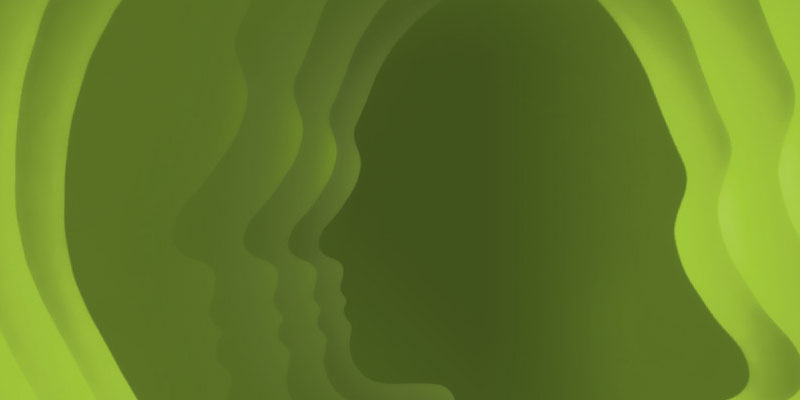
An Excess of Positive Results: Comparing the Standard Psychology Literature With Registered Reports
Anne M. Scheel, Mitchell R. M. J. Schijen, and Daniël Lakens
When the only results published are those that support the tested hypotheses (i.e., “positive” results), evidence for scientific claims is distorted. Scheel and colleagues compared the results published in Registered Reports (RRs)—a new publication format in which authors commit to peer review and publishing before the results are known—with a random sample of results reported in standard publications. They found that 44% of results in RRs are positive, compared with 96% in standard publications. Scheel and colleagues suggest that there might be reduced publication bias and/or inflation of Type I error (i.e., rejection of a true null hypothesis) in RRs.
Psychologists Should Use Brunner-Munzel’s Instead of Mann-Whitney’s U Test as the Default Nonparametric Procedure
Julian D. Karch
Mann-Whitney’s U test makes fewer assumptions than its parametric alternative, the t test, and, for instance, can be used when the data are ordinal. However, Mann-Whitney’s test still makes strong assumptions. Karch suggests that these assumptions are not frequently met in psychology, which can invalidate the test and lead to rejections of true null hypotheses, even for large samples. To address this, Karch introduces Brunner-Munzel’s test, which has similar power as Mann-Whitney’s test but provides more valid results, even when certain assumptions are violated. The author explains how to perform and report Brunner-Munzel’s test.
Improving Transparency, Falsifiability, and Rigor by Making Hypothesis Tests Machine-Readable
Daniël Lakens and Lisa M. DeBruine
Lakens and DeBruine propose an approach to make hypothesis tests machine-readable. Specifying hypothesis tests in ways that a computer can read and evaluate might increase the rigor and transparency of hypothesis testing as well as facilitate finding and reusing these tests and their results (e.g., in meta-analyses). The authors describe what a machine-readable hypothesis test should look like and demonstrate its feasibility in a real-life example (DeBruine’s 2002 study on facial resemblance and trust), using the prototype R package scienceverse.
Evaluating Response Shift in Statistical Mediation Analysis
A. R. Georgeson, Matthew J. Valente, and Oscar Gonzalez
In intervention research, researchers target intermediate variables (mediators) thought to be related to an outcome and measure the changes in those variables. Mediators are often measured using participants’ self-reports, which can lead to response shifts (e.g., participants in a treatment group might reinterpret the mediator and, therefore, recalibrate their responses). Thus, changes in mediators across groups or time might reflect a combination of true change and response shift. Georgeson and colleagues provide background on the theory and methodology used to detect response shift (i.e., tests of measurement invariance) and a simulation of the effects of response shift.
Precise Answers to Vague Questions: Issues With Interactions
Julia M. Rohrer and Ruben C. Arslan
Rohrer and Arslan discuss issues regarding the prediction and interpretation of interactions (when one variable’s effect depends on another variable). They suggest that being aware of these issues can help researchers choose the correct analyses for their research questions. First, interactions can appear or disappear depending on scaling decisions; second, interactions may be conceptualized as changes in slope or changes in correlations; and third, interactions may or may not be causally identified. Rohrer and Aslan provide examples of these issues and recommendations for how to address them.
How Do We Choose Our Giants? Perceptions of Replicability in Psychological Science
Manikya Alister, Raine Vickers-Jones, David K. Sewell, and Timothy Ballard
Alister and colleagues surveyed the corresponding authors of articles published between 2014 and 2018 regarding 76 study attributes that might affect the replicability of a finding. Six types of features appeared to heavily influence the degree of confidence researchers had in the replicability of findings. These features were related to: weak methodology (e.g., low power) and lack of transparency, questionable research practices, rigorous analyses (e.g., large sample), ease of conducting a replication (e.g., the existence of previous replications, open data, or open methods), robustness of the findings (e.g., consistency with theory), and traditional markers of replicability (e.g., status of the researcher or institution).
A Guide to Posting and Managing Preprints
Hannah Moshontz, Grace Binion, Haley Walton, Benjamin T. Brown, and Moin Syed
Moshontz and colleagues provide a guide to help researchers post unpublished versions of their work and manage these preprints before, during, and after the peer-review process to achieve different goals (e.g., get feedback, speed dissemination). Their recommendations include posting preprints in a dedicated server that assigns DOIs, provides editable metadata, is indexed by Google Scholar, supports review and endorsements, and supports version control. They also suggest including the draft date and information about the article’s status on the cover page and licensing preprints in a way that allows public use with attribution.
Leveraging Containers for Reproducible Psychological Research
Kristina Wiebels and David Moreau
The use of containers to isolate computer environments and virtualize operating systems is a lightweight alternative to virtual machines that also lets researchers recreate computing environments used by other researchers. Containerization might support greater reproducibility by allowing researchers to examine and replicate previous research in the exact same conditions in which it was originally conducted, regardless of installed software, drivers, and operating systems. In this tutorial, Wiebels and Moreau explain what containers are and the problems they might solve. They provide step-by-step examples of the implementation of containerization using Docker and R.
APS regularly opens certain online articles for discussion on our website. Effective February 2021, you must be a logged-in APS member to post comments. By posting a comment, you agree to our Community Guidelines and the display of your profile information, including your name and affiliation. Any opinions, findings, conclusions, or recommendations present in article comments are those of the writers and do not necessarily reflect the views of APS or the article’s author. For more information, please see our Community Guidelines.
Please login with your APS account to comment.