Featured
I, Psychologist: Exploring the Ethical Hurdles and Clinical Advantages of AI in Healthcare
Patients are often resistant to the use of artificial intelligence in healthcare. But if their concerns are taken to heart, AI-assisted care could usher in a new era of personalized medicine.
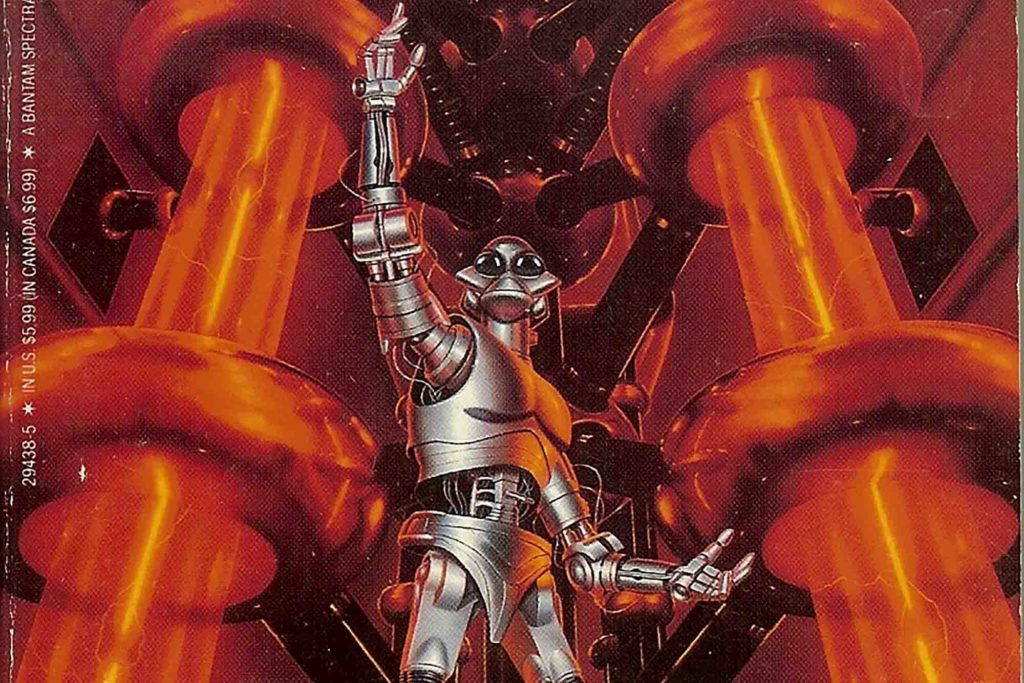
Image above: Isaac Asimov – I, Robot” by RA.AZ is licensed under CC BY 2.0. The collection was first published in 1950.
- Collecting longitudinal data from wearables, social media, and other sources could help paint a clearer picture of individual patients’ well-being in the “clinical whitespace” between appointments.
- Patients’ concerns about “uniqueness neglect”—the fear that AI will overlook an individual’s specific symptoms and circumstances—can be alleviated by emphasizing AI’s ability to tailor care to each patient’s characteristics.
- In order for underrepresented patients to benefit equally from AI-assisted healthcare, developers must go out of their way to include diverse samples in their datasets and to understand how each AI technology is making decisions.
- Predictive algorithms are highly accurate at the group level and can be used to identify which psychological interventions a patient is most likely to benefit from.
- AI’s predictive potential can be enhanced by using a person-specific approach to capture the true complexity of human behavior.
The public’s longstanding anxiety about artificial intelligence (AI) in our daily lives is reflected in countless science-fiction horror stories about wayward androids and killer smart homes, as well as in the work of renowned sci-fi author Isaac Asimov. Many of his classic short stories, such as those featured in his 1950 collection I, Robot, explore how AI—if restrained by three theoretical rules of robotics (a robot must not injure a human being, must obey orders, and must protect its own existence)—might serve or circumvent humanity.
Some of these stories even touch upon how AI technologies could influence the development of human healthcare. In The Bicentennial Man, Asimov’s 1976 short story, which was later adapted into a movie starring Robin Williams, the author imagined an independently operated robotic surgeon as a machine so single-mindedly specialized that “there would be no hesitation in his work, no stumbling, no quivering, no mistakes,” he wrote.
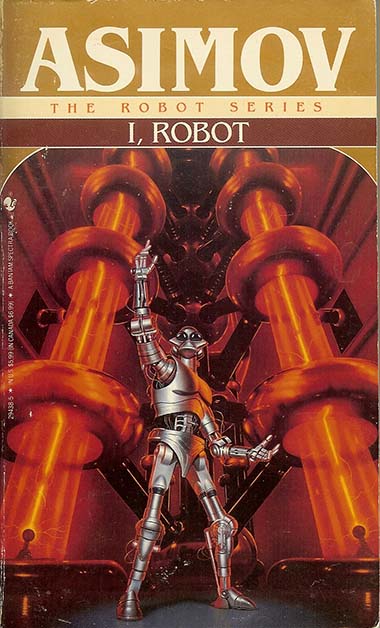
Even if such precisely automated surgery were proven to be more effective than a human surgeon, however, research suggests that the fear of “uniqueness neglect”—being treated as just another cog in an AI’s medical machinery—could make many patients resistant to being diagnosed by AI, much less to going under its automated knife.
“The prospect of being cared for by AI providers is more likely to evoke a concern that one’s unique characteristics, circumstances, and symptoms will be neglected,” wrote Chiara Longoni (Boston University), Andrea Bonezzi (New York University), and APS Fellow Carey K. Morewedge (Boston University) in a 2019 Journal of Consumer Research article. “Consumers view machines as capable of operating only in a standardized and rote manner that treats every case the same way.”
Through a series of 11 surveys involving more than 2,500 participants recruited from university campuses and Amazon Mechanical Turk, Longoni and colleagues found that people were less likely to schedule, and less willing to pay for, a hypothetical automated diagnostic exam than an exam with a human provider, even when the two were explicitly presented as equally accurate. Participants who reported perceiving themselves as more unique were found to be even more resistant to receiving automated care and less likely to follow through on the AI medical recommendations.
Fortunately, Longoni and colleagues also found that when AI was presented as providing “personalized care” or as supporting, rather than replacing, a human caregiver, patients became just as likely to accept automated care as that of a human doctor.
“Personalized medicine appears to curb resistance to medical AI because it reassures consumers that care is tailored to their own unique characteristics, thus assuaging uniqueness neglect,” Longoni and colleagues wrote.
In the context of mental healthcare, AI technology can also offer practitioners new insight into the day-to-day well-being of their patients, supporting the use of more effective interventions.
Measuring well-being in the moment
Integrating digital life data from wearables, apps, and social media into therapeutic work can help fill in the “clinical whitespace” between appointments, wrote Glen Coppersmith, the chief data officer at the therapy company SonderMind, in a 2022 Current Directions in Psychological Science article. Traditional clinical measures rely on patients being able to accurately report their past feelings and behavior through surveys and journaling, Coppersmith explained, but allowing patients to opt in to digital life-data collection could provide clinicians with a wealth of passive, longitudinal data about fluctuations in well-being that patients might not even be aware of themselves.
“Unlike a broken bone, which is broken regardless of where you are, mental health is almost by definition what is happening outside of the therapist’s office, where the client interacts with the real world,” Coppersmith said in an interview. “There is good evidence that when we do incorporate measurement-based care, outcomes improve. … This is just a different, broader approach to what we are measuring.”
Machine learning could help identify patterns in these data, he suggested, allowing AI to prompt therapists to check in on their patients early in a depressive episode or psychotic break, for example. These alerts could also be used to encourage patients to take action when their mental health takes a turn for the worse, even going so far as to provide “just-in time” interventions to people at risk of attempting suicide.
“It holds the potential for profound change, including a better understanding of what works for whom, leading to more personalized self-care and therapeutic care, more effective use of therapists’ time, and more continuous instead of point-in-time measurement of how someone is doing,” Coppersmith said.
There is also good evidence that this kind of measurement-based care improves patient outcomes, he added.
Predictive modeling enhanced by machine learning, for example, could help practitioners select more effective treatments for chronic mental health conditions according to their patients’ unique characteristics. In a 2022 article in Clinical Psychological Science, Zachary D. Cohen (University of California, Los Angeles) and colleagues used 2 years of retrospective data to predict whether patients with clinical depression would experience better outcomes by remaining on their current course of antidepressants or receiving additional mindfulness-based cognitive behavioral therapy (MBCT). When patients who were predicted to be at high risk of a depression relapse were also given MBCT, they were 22% less likely to experience a relapse than if they were left on antidepressants alone.
But although predictive modeling informed by digital life data may open a new window into a patient’s state of mind, using and storing such sensitive information requires seriously considering the implications, Coppersmith acknowledged. Assuring patients that AI use of their data would be opt-in only could help alleviate concerns about patient consent, but that data must be stored securely to preserve their privacy.
For industry data scientists like Coppersmith, addressing these concerns may primarily entail engaging with therapists to determine what they need to feel comfortable integrating digital life data into their patient care.
Algorithms can be biased too
Practitioners and researchers alike have already given considerable thought to addressing the ethical pitfalls of using AI in mental healthcare, but work remains to be done. For example, although AI can be used to help keep practitioners’ implicit biases in check, AI can also be biased against patients of minority racial, ethnic, and cultural backgrounds if the dataset includes too few people from those populations, noted Coppersmith in his Current Directions in Psychological Science article. Certain algorithms, for example, have been shown to be less accurate at identifying depression in underrepresented groups. In order for these patients to benefit equally from the use of AI, he added, they need to be represented in the training data used to generate predictions.
Medical AI is at risk of being influenced by patients’ identities even when it is not intentionally given access to that information. In a 2022 Lancet Digital Health study, Judy Wawira Gichoya (Emory University School of Medicine) and colleagues found that AI can accurately predict patients’ race from x-ray images, something human doctors are not able to do themselves. If practitioners are going to use image-based AI to make decisions about patient care, researchers need to understand how the AI is determining patients’ race so that this doesn’t unintentionally influence its recommendations, Gichoya and colleagues explained.
The interplay of human and algorithmic bias can be observed in our criminal justice systems, noted APS fellow Robert L. Goldstone (Indiana University) in his introduction to the 2022 Current Directions in Psychological Science special issue on behavioral measurement. On the one hand, research has shown that judges, when making decisions without recommendations from an AI technology, are more likely to reject requests for asylum when the weather is hot, resulting in arbitrarily unequal application of immigration law and demonstrating. On the other hand, risk-assessment algorithms have been shown to falsely predict that Black defendants would commit another crime at nearly twice the rate of White defendants, leading to harsher sentencing along racial lines.
This demonstrates that, despite AI’s potential to limit the impact of powerful individuals’ mood or prejudices on what should be impartial decisions, this technology is not immune to the biases of the society that created it.
“At the societal level, the potential benefits of reducing bias and decision variability by using objective and transparent assessments are offset by threats of systematic, algorithmic bias from invalid or flawed measurements,” wrote Goldstone. “Considerable technological progress, careful foresight, and continuous scrutiny will be needed so that the positive impacts of behavioral measurement technologies far outweigh the negative ones.”
Related content we think you’ll enjoy
-
Children, Creativity, and the Real Key to Intelligence
APS President Alison Gopnik writes that the contrast between the reasoning of creative 4-year-olds and predictable artificial intelligence may be a key to understanding how human intelligence works.
-
Me, My Job, and AI: Preserving Worker Identity Amid Technological Change
How artificial intelligence is functionally deployed in the workplace impacts whether workers feel threatened by it or embrace it.
-
A Cloudy Future: Why We Don’t Trust Algorithms When They’re Almost Always Right
Researchers explore our preference for human skill and instinct over technologies that have proven themselves better than us at driving, performing surgery, and making hiring decisions.
A person-specific approach to human behavior
Capturing data from diverse populations is important for improving patient outcomes at the group level, but predicting individual behavioral outcomes requires a person-specific approach, said Emorie D. Beck (Northwestern University Feinberg School of Medicine; University of California, Davis) in an interview. Drilling down to the individual level allows the predictions generated by statistical models (including machine learning) to reflect the true complexity of human behavior, including how specific people may react to an intervention.
“When we’re doing group-level prediction, we’re talking about how situations differ and assuming that we can come to some sort of average prediction of what a person with characteristics like this in situations like this would do, whereas in a person-specific framework we don’t assume as much,” said Beck. “People who are seemingly similar can react differently to the same situations.”
Beck and Joshua J. Jackson (Washington University in St. Louis) investigated the extent to which individuals’ personality, mood, and past responses to similar situations could predict their future loneliness, procrastination, and study habits through a longitudinal study of 104 university students. Participants completed an average of 57 assessments between October 2018 and December 2019 detailing how their self-reported personality and mood related to what they had been doing in the past hour.
Through comparing the accuracy of multiple machine learning algorithms, Beck and Jackson found that both personality and situational factors predicted individuals’ loneliness, procrastination, and studying—but exactly which personality and situational factors predicted these behaviors, and to what extent, varied significantly between participants. The most common relationship, for example, was between reported participants’ energy levels and their likelihood of arguing with a friend or family member, but just 40% of participants shared these, and no two participant profiles included exactly the same factors in their personalized models.
“Individual differences reigned supreme—people differed on how predictable outcomes were, which domains performed best, and which features were most important,” Beck and Jackson wrote.
Taking a precision-medicine approach to psychological assessment could help that complexity shine through, Beck said. In an upcoming study, Beck will also explore how the predictive power of assessments could be improved by asking participants to generate their own items on a survey. This could help researchers identify risk factors for behaviors that they may not have considered before, she said.
“When we think about doing some of this precision, personalized medicine ethically, it’s going to require some really close attention to the people we’re working with, and also the communities that they’re embedded in,” Beck said. “We have to treat people as stakeholders in their own health and well-being, because if we don’t they become these cogs that we get to manipulate, and we can lose sight of the very real consequence that any intervention can have for people.”
Feedback on this article? Email [email protected] or login to comment. Interested in writing for us? Read our contributor guidelines.
Asimov, I. (1976). The bicentennial man. Ballantine Books.
Beck, E. D., & Jackson, J. J. (2022). Personalized prediction of behaviors and experiences: An idiographic person–situation test. Psychological Science, 33(10), 1767–1782. https://doi.org/10.1177/09567976221093307
Cohen, Z. D., DeRubeis, R. J., Hayes, R., Watkins, E. R., Lewis, G., Byng, R., Byford, S., Crane, C., Kuyken, W., Dalgleish, T., & Schweizer, S. (2022). The development and internal evaluation of a predictive model to identify for whom mindfulness-based cognitive therapy offers superior relapse prevention for recurrent depression versus maintenance antidepressant medication. Clinical Psychological Science. https://doi.org/10.1177/21677026221076832
Coppersmith, G. (2022). Digital life data in the clinical whitespace. Current Directions in Psychological Science, 31(1), 34–40. https://doi.org/10.1177/09637214211068839
Gichoya, J. W., Banerjee, I., Bhimireddy, A. R., Burns, J. L., Celi, L. A., Chen, L. C., Correa, R., Dullerud, N., Ghassemi, M., Huang, S. C., Kuo, P. C., Lungren, M. P., Palmer, L. J., Price, B. J., Purkayastha, S., Pyrros, A. T., Oakden-Rayner, L., Okechukwu, C., Seyyed-Kalantari, … Zhang, H. (2022). AI recognition of patient race in medical imaging: A modelling study. Lancet Digital Health, 4, Article e406–14. https://doi.org/10.1016/S2589-7500(22)00063-2
Goldstone, R. L. (2022). Performance, well-being, motivation, and identity in an age of abundant data: Introduction to the “well-measured life.” Current Directions in Psychological Science, 31(1), 3–11. https://doi.org/10.1177/09637214211053834
Longoni, C., Bonezzi, A., & Morewedge, C. K. (2019). Resistance to medical artificial intelligence. Journal of Consumer Research, 46(4), 629–650. https://doi.org/10.1093/jcr/ucz013
APS regularly opens certain online articles for discussion on our website. Effective February 2021, you must be a logged-in APS member to post comments. By posting a comment, you agree to our Community Guidelines and the display of your profile information, including your name and affiliation. Any opinions, findings, conclusions, or recommendations present in article comments are those of the writers and do not necessarily reflect the views of APS or the article’s author. For more information, please see our Community Guidelines.
Please login with your APS account to comment.