New Research in Psychological Science
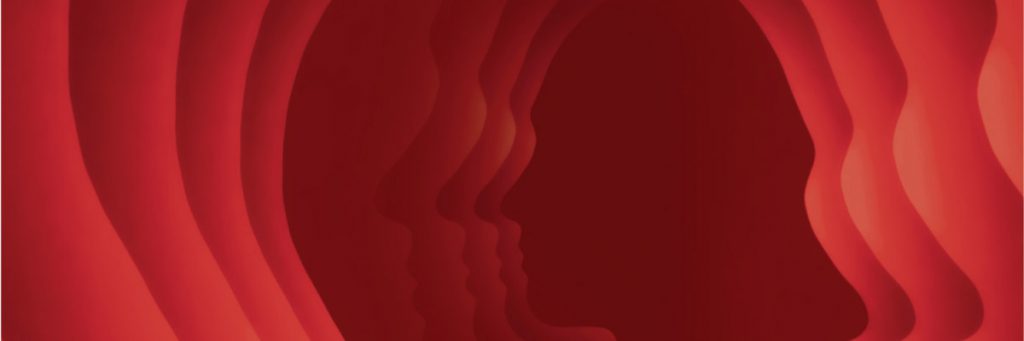
Racial Bias in Police Traffic Stops: White Residents’ County-Level Prejudice and Stereotypes Are Related to Disproportionate Stopping of Black Drivers
Marleen Stelter, Iniobong Essien, Carsten Sander, and Juliane Degner
Stelter and colleagues investigated the link between racial disparities in police traffic stops and regional racial bias, using data from more than 130 million police traffic stops in 1,413 U.S. counties and county-level measures of racial bias from more than 2 million online respondents. In the majority of counties, Black drivers were stopped at rates disproportionate to their population share of county demographics. Disproportionate stopping of Black drivers was higher in counties where White residents expressed more racial prejudice. The relationships between regional-level bias and racial disparities in policing highlight the importance of the context in which police operate.
Racial Demographics Explain the Link Between Racial Disparities in Traffic Stops and County-Level Racial Attitudes
Pierce D. Ekstrom, Joel M. Le Forestier, and Calvin K. Lai
Ekstrom and colleagues found evidence for a relationship between racial disparities in traffic stops and racial demographics. Like Stelter and colleagues, they used data from the Stanford Open Policing Project and corresponding county-level data on implicit and explicit racial attitudes from the Project Implicit research website, finding that racial demographics helped explain the association between Black–White traffic-stop disparities and county-level implicit and explicit racial attitudes. That is, counties with higher proportions of White residents had larger racial disparities in police traffic stops. Although racial disparities in police stop outcomes (e.g., arrest rates) existed, these did not appear to be systematically related to racial attitudes.
Explaining the Spatial Patterning of Racial Disparities in Traffic Stops Requires a Structural Perspective: Further Reflections on Stelter et al. (2022) and Ekstrom et al. (2022)
B. Keith Payne and Julian M. Rucker
In this invited Further Reflections article, Payne and Rucker analyze the articles by Stelton et al. and Ekstrom et al., whose results challenge the idea that the explanation for racial disparities in policing is “a few bad apples” responsible for stopping Black drivers. Instead, they say, the results call for explanations at the level of communities, a factor that both research articles suggest is linked to their findings, though they do not deeply explore it. Payne and Rucker argue that answering this question may require going beyond the two data sets to consider structural inequalities and the structural factors associated with the predictors (implicit and explicit bias, population demographics) and the outcomes (policing).
The Importance of Random Slopes in Mixed Models for Bayesian Hypothesis Testing
Klaus Oberauer
Previous research has shown that excluding random slopes—differences between individuals in an effect’s direction and size—from a frequentist mixed model can lead to a substantial increase in false-positive conclusions. Here, Oberauer demonstrated through five simulations that the same is true for Bayesian hypothesis testing with mixed models. Including random slopes largely eliminates the risk of strong false positives but reduces the chance of obtaining strong evidence for true effects. He recommends starting analysis by testing the support for random slopes in the data and removing them from the models only if there is clear evidence against them.
Learning About the Self: Motives for Coherence and Positivity Constrain Learning From Self-Relevant Social Feedback
Jacob Elder, Tyler Davis, and Brent L. Hughes
People learn about themselves from social feedback, but desires for coherence and positivity constrain how they incorporate feedback into their self-concepts, this research suggests. Participants evaluated themselves on a network of personality traits while receiving both positive and negative social feedback from other people. Overall, participants adjusted their self-views according to others’ feedback but disproportionately incorporated positive feedback more than negative feedback. They also resisted changing their self-views of traits with more perceived implications, which may help to preserve their sense of coherence. However, people with worse mental health and well-being incorporated less positive than negative feedback, indicating that mental health might relate to asymmetrical self-learning.
Motivating Personal Growth by Seeking Discomfort
Kaitlin Woolley and Ayelet Fishbach
Wooley and Fishbach suggest that seeking discomfort as a signal of growth can increase motivation. They tested this prediction across various areas of personal growth: taking improvisation classes to increase self-confidence, engaging in expressive writing to process difficult emotions, becoming informed about the COVID-19 health crisis, opening oneself to opposing political viewpoints, and learning about gun violence. Across the areas of personal growth that caused immediate discomfort, instructions to seek discomfort as a signal of self-growth motivated engagement and increased perceived goal achievement more than standard instructions did. Thus, embracing discomfort can be motivating.
Illusory Feelings, Elusive Habits: People Overlook Habits in Explanations of Behavior
Asaf Mazar and Wendy Wood
This research suggests that people may overlook the influence of habits, preferring to attribute their behavior to inner states, such as mood. Mazar and Wood tested this misattribution in an experiment examining helping behavior as well as in an ecological momentary assessment study of college students’ everyday coffee drinking. Both studies indicated that habit strength predicted behavior better than inner states did. However, participants’ explanations of their behavior emphasized their inner states, even when they were incentivized for accuracy and asked to explain other people’s behavior. Overlooking habits in this way may lead to poor self-regulation of habitual behavior.
Globally Inaccurate Stereotypes Can Result From Locally Adaptive Exploration
Xuechunzi Bai, Susan T. Fiske, and Thomas L. Griffiths
Bai and colleagues found that globally inaccurate stereotypes about social groups can result from locally adaptive exploration. That is, to maximize the long-term rewards of interactions, people use past interactions to choose whom to interact with. Early positive experiences with some groups may discourage them from investigating other groups that could yield equally positive experiences. The researchers developed a model and conducted two experiments in which participants met people from different fictitious groups and decided how to interact with them. Choosing among groups to maximize the long-term benefit of interactions, a natural adaptive choice, was enough to produce inaccurate assessments of different groups.
Eliminating the Low-Prevalence Effect in Visual Search With a Remarkably Simple Strategy
J. Eric T. Taylor, Matthew D. Hilchey, Blaire J. Weidler, and Jay Pratt
The low-prevalence effect in visual search occurs when rare targets are missed at a disproportionately high rate. This effect can pose serious harm in applied settings (e.g., in medical imaging, rare signs of cancer may go undetected). Taylor and colleagues document a reduction of the effect using a simple change of instructions. Instead of asking participants to search for the presence or absence of a target, they asked participants to engage in “similarity search”—to identify the display element most similar to a target on every trial, regardless of whether a target was present.
Feedback on this article? Email [email protected] or post a comment.
APS regularly opens certain online articles for discussion on our website. Effective February 2021, you must be a logged-in APS member to post comments. By posting a comment, you agree to our Community Guidelines and the display of your profile information, including your name and affiliation. Any opinions, findings, conclusions, or recommendations present in article comments are those of the writers and do not necessarily reflect the views of APS or the article’s author. For more information, please see our Community Guidelines.
Please login with your APS account to comment.