New Content from Advances in Methods and Practices in Psychological Science
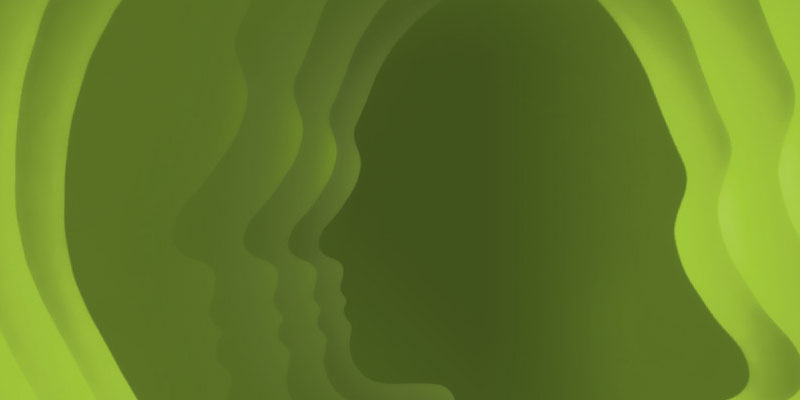
Multilab Direct Replication of Flavell, Beach, and Chinsky (1966): Spontaneous Verbal Rehearsal in a Memory Task as a Function of Age
Emily M. Elliott et al.
In 1966, Flavell, Beach, and Chinsky found that children 5 to 6 years old seldom verbalized during a short-term memory task, but verbalizations increased after that age. These findings have been interpreted as showing that children younger than 7 do not produce verbalizations that might aid memory. Elliot and colleagues attempted to replicate these findings across 17 labs. They found instead that a substantial portion of 5- and 6-year-olds produced verbalizations at least sometimes during a memory task (and thus performed better in the task), and there was no continuous increase in verbalization from 7 to 10 years old. These findings are significant for theories of cognitive development.
ManyClasses 1: Assessing the Generalizable Effect of Immediate Feedback Versus Delayed Feedback Across Many College Classes
Emily R. Fyfe et al.
Fyfe and colleagues introduce a model to establish an experimental paradigm for evaluating the benefits of recommended educational practices in authentic educational contexts, beyond the lab. With ManyClasses, researchers examine the same experimental effect across many classes, focusing on different topics, institutions, teacher implementations, and student populations. Here, the researchers evaluated whether the timing of feedback on class assignments affected subsequent student performance. Results indicated that, across 38 classes, there were no overall differences between the effect of immediate feedback versus delayed feedback on student performance. However, data suggested that delayed feedback might modestly outperform immediate feedback in certain classes.
A Primer on Bayesian Model-Averaged Meta-Analysis
Quentin F. Gronau, Daniel W. Heck, Sophie W. Berkhout, Julia M. Haaf, and Eric-Jan Wagenmakers
Gronau and colleagues discuss an alternative to frequentist meta-analysis—Bayesian model-averaged meta-analysis. In Bayesian model-averaged meta-analysis, researchers combine the results of four Bayesian meta-analysis models: fixed-effect null hypothesis, fixed-effect alternative hypothesis, random-effects null hypothesis, and random-effects alternative hypothesis. Given the data, each of these models has different plausibilities to address whether the overall effect is different from zero and whether there is between-study variability in effect size. By combining the models according to these plausibilities, Bayesian model-averaged meta-analysis takes into account model uncertainty and avoids the need to select a fixed-effect or a random-effects model.
Australian and Italian Psychologists’ View of Replication
Franca Agnoli, Hannah Fraser, Felix Singleton Thorn, and Fiona Fidler
Agnoli and colleagues surveyed Australian and Italian researchers about the meaning and role of replication studies. Almost all participants (98% of Australians and 96% of Italians) defined replication as direct replication (i.e., using the exact same method as in the original experiment). Most participants agreed that replications are important in psychology, should be conducted and published more often, and should be adequately funded. The most frequently mentioned obstacle to replications was the difficulty of publishing them, although participants also overestimated by two to three times the percentage of studies in psychology that are replications (5%).
Summary Plots With Adjusted Error Bars: The superb Framework With an Implementation in R
Denis Cousineau, Marc-André Goulet, and Bradley Harding
In figures showing data, error bars conveying confidence intervals provide limited information about the precision of estimated results. For instance, confidence intervals do not allow the reader to compare results between groups, between repeated measures, when participants are clustered, and when the population size is finite. Thus, inferences from such error bars can be at odds with conclusions derived from statistical tests. Here, Cousineau and colleagues propose adjusting confidence intervals so that they reflect the experimental design and sampling strategy used. To facilitate the creation of plots with error bars reflecting the adjustments, the researchers developed superb, an open-source library for R.
Putting Psychology to the Test: Rethinking Model Evaluation Through Benchmarking and Prediction
Roberta Rocca and Tal Yarkoni
How should we evaluate models and theories in psychological science? Rocca and Yarkoni suggest that introducing common benchmarks to evaluate psychological science may foster cumulative progress and encourage researchers to consider the practical utility of scientific models. The researchers draw inspiration from fields such as machine learning and provide guidelines and concrete suggestions on how to develop these common benchmarks (each consists of a data set of coded examples and a task specification defining the metrics that will be used to quantify the model’s predictions). Rocca and Yarkoni also address potential concerns that may arise during the development of benchmarks.
Citation Patterns Following a Strongly Contradictory Replication Result: Four Case Studies From Psychology
Tom E. Hardwicke et al.
Hardwicke and colleagues examined the citation patterns that followed four multilaboratory replication attempts that contradicted or outweighed the original findings. Results indicated that a published replication led immediately to a small decrease of favorable citations of the original article and a small increase of unfavorable citations of the original article. These results suggest a perpetuation of belief in the original findings and only a modest corrective effect. Moreover, the replication studies were not cited as often as the original research in new articles. Thus, it appears that replication results that contradict original findings might not prompt a corrective response from the research community.
Doing Better Data Visualization
Eric Hehman and Sally Y. Xie
In this tutorial, Hehman and Xie focus on how to design data visualizations as clearly as possible. The tutorial is accessible to both experienced and relatively new users of R and the R package ggplot2, but users should have some basic statistical and visualization knowledge, the authors advise. Hehman and Xie cover guiding philosophies (e.g., minimalism, color choice) and knowledge about the science of data visualization, available in books, blogs, and online discussion forums. Based on this knowledge, they offer recommendations and specific examples of code in R for visualizing central tendencies, proportions, and relationships between variables.
A Guide to Visualizing Trajectories of Change With Confidence Bands and Raw Data
Andrea L. Howard
In this tutorial, Howard provides a guide to visualize trajectories of change over time. The tutorial is intended for researchers who are already modeling data over time using random-effects regression or latent curve modeling but need a more comprehensive guide or want to make graphics using R. Howard uses an example of plotting trajectories from two groups, as in random-effects models that include Time x Group interactions and latent curve models that regress the talent time slope factor onto a grouping variable. Prior knowledge of R is not needed to use the tutorial, and readers can find all the supporting materials at https://osf.io/78bk5/.
Feedback on this article? Email [email protected] or comment below.
APS regularly opens certain online articles for discussion on our website. Effective February 2021, you must be a logged-in APS member to post comments. By posting a comment, you agree to our Community Guidelines and the display of your profile information, including your name and affiliation. Any opinions, findings, conclusions, or recommendations present in article comments are those of the writers and do not necessarily reflect the views of APS or the article’s author. For more information, please see our Community Guidelines.
Please login with your APS account to comment.