A Cloudy Future: Why We Don’t Trust Algorithms When They’re Almost Always Right
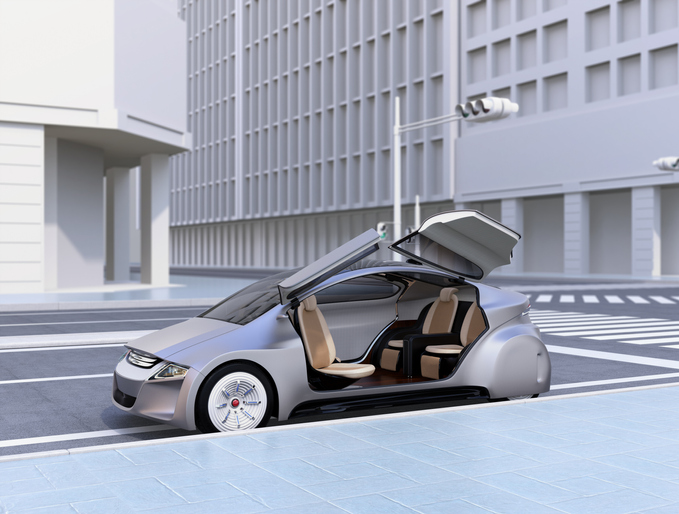
Roomba sales are soaring, suggesting that millions of people trust the robotic vacuums’ room-navigation algorithms and powerful suction power to keep their floors clean. Similarly, few computer users object to the statistical models and other algorithms that help us conduct web searches, see relevant social media posts, and get recommendations from Netflix and Spotify.
But are we ready to embrace algorithms for activities with less-certain outcomes and arguably higher stakes—such as selecting investments, driving cars, performing surgery, or assessing job and university applications?
Not yet, according to new research in Psychological Science.
“These results suggest that convincing people to use algorithms in inherently uncertain domains is not a case of waiting until technology improves and algorithms perform better than they do today.”
Berkeley J. Dietvorst and Soaham Bharti (University of Chicago)
“To the extent that investing, medical decision-making, and other domains are inherently uncertain, people may be unwilling to use even the best possible algorithm in those domains,” wrote Berkeley J. Dietvorst and Soaham Bharti (University of Chicago). This is despite the fact that algorithmic forecasters outperform human forecasters in these domains, thanks to their use of tools that follow more consistent rules and make fewer errors than humans do.
“This unwillingness to adopt algorithms that outperform humans can have an enormous cost,” the researchers continued. For example, “the majority of Americans report that they would not be comfortable riding in a self-driving car…but research suggests that early adoption of self-driving cars could save hundreds of thousands of lives.”
What explains our preference for human skill and instinct over technologies that have proven themselves better than us at driving, performing surgery, and making hiring decisions? In essence, we trust ourselves to take risks on decisions that are irreducibly uncertain, but we don’t trust computers to take such risks for us because their errors seem more pronounced on the very rare occasions they occur.
“We propose that people have diminishing sensitivity to forecasting error, which causes them to prefer decision-making methods that they believe have the highest likelihood of providing a near-perfect answer (i.e., one with little to no error),” Dietvorst and Bharti wrote. “This decision strategy encourages risk-taking and results in people favoring riskier, and often worse-performing, decision-making methods (such as human judgment) when they feel that an algorithm is unlikely to generate a near-perfect answer.”
In nine studies, the researchers showed that people perceive “relatively large subjective differences between different magnitudes of near-perfect forecasts (the best possible forecasts that produce little to no error) and relatively small subjective differences between forecasts with greater amounts of error.” As a result, they are less likely to choose the best decision-makers in domains that are more unpredictable (e.g., with random outcomes vs. with outcomes determined by an equation) and instead tend to prefer decision-makers based on their perceived likelihood of producing a near-perfect choice and with high variance in performance. This leads people to favor riskier and often worse-performing decision-makers, such as human judgment, in uncertain domains.
“These results suggest that convincing people to use algorithms in inherently uncertain domains is not a case of waiting until technology improves and algorithms perform better than they do today,” the researchers concluded. “The impact of this refusal is substantial, as society will not fully benefit from technological progress in consequential but uncertain domains until people are willing to use algorithms to make inherently uncertain predictions.”
But Do Machines Have Ethics?
Another recent article explores alarm over so-called driverless dilemmas, in which autonomous vehicles must make high-stakes ethical decisions on the road, such as whom to harm and whom to save (e.g., a pedestrian or a passenger). These concerns are an engineering and policy distraction, according to Julian De Freitas and others. “We do not teach humans how to drive by telling them whom to kill if faced with a forced choice. This is because planning for an unlikely, undetectable, and uncontrollable situation would be a distraction from the goal we do teach novice drivers: minimize harming anyone.” The same goal should apply to self-driving cars, the researchers said.
References
Alvarez, G. A., Anthony, S. E., Censi, A., & De Freitas, J. (2020). Doubting Driverless Dilemmas. Perspectives on Psychological Science, 15(5),1284-1288. https://doi.org/10.1177/1745691620922201
Bharti, S., & Dietvorst, B. J. (2020). People Reject Algorithms in Uncertain Decision Domains Because They Have Diminishing Sensitivity to Forecasting Error. Psychological Science, 31(10), 1302-1314. https://doi.org/10.1177/0956797620948841
APS regularly opens certain online articles for discussion on our website. Effective February 2021, you must be a logged-in APS member to post comments. By posting a comment, you agree to our Community Guidelines and the display of your profile information, including your name and affiliation. Any opinions, findings, conclusions, or recommendations present in article comments are those of the writers and do not necessarily reflect the views of APS or the article’s author. For more information, please see our Community Guidelines.
Please login with your APS account to comment.