No Crisis but No Time for Complacency
Coming to Consensus on Reproducibility
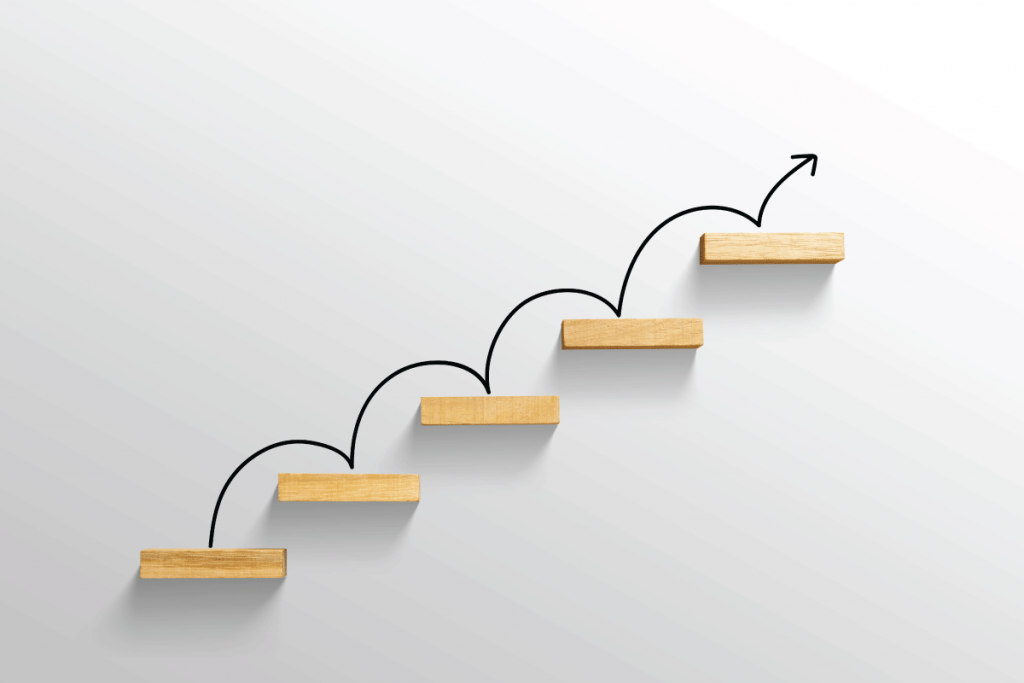
The National Academies of Sciences, Engineering, and Medicine recently published a report titled Reproducibility and Replicability in Science. We both had the privilege of serving on the committee that issued the report, and this is a brief summary of how the committee came about and its main findings.
In response to concerns about replicability in many branches of science, Congress — via the National Science Foundation — directed the National Academies to conduct a study. The mandate was broad: to define reproducibility and replicability, assess what is known about how science is doing in these areas, review current attempts to improve reproducibility and replicability, and make recommendations for improving rigor and transparency in research — across all fields of science and engineering, not just psychological science.
A committee of 13 scientists was formed that, in addition to us, included geoscientists, medical researchers, natural scientists, engineers, computer scientists, historians of science, and statisticians. The committee met 12 times in a period of 16 months. This was not too difficult for Tim, who could hop on a train in Charlottesville and be in Washington in a couple of hours. It was more difficult for Wendy, who interspersed a sabbatical in Paris with flying back and forth to DC several times. Regardless, we both agree that it was a fascinating and enlightening experience to serve on the committee.
So, what did the committee conclude? Our job was first to define reproducibility and replicability. As you can imagine, definitions vary greatly across disciplines, and our consensus definitions were hammered out from a range of possibilities.
We defined reproducibility as computational reproducibility — obtaining consistent computational results using the same input data, computational steps, methods, code, and conditions of analysis. Replicability was defined as obtaining consistent results across studies that were aimed at answering the same scientific question, each of which obtained its own data. In short, reproducing research involves using the original data and code, whereas replicating research involves new data collection and methods similar to those used in previous studies.
Once we defined our terms, what did the committee conclude about the state of reproducibility and replicability in science? This question is probably foremost in many people’s minds, given the attention it has received, both in our field and in the national media. And, as anyone who has followed this debate knows, there is considerable disagreement about the answer. Some believe that our field faces severe problems, such as frequent use of lax methods, that threaten validity. Others feel that the extent of these problems has been exaggerated. Still other researchers note that rigorous research practices have been an important focus in psychological science and other scientific fields long before the current concerns with reproducibility and replicability.
The committee’s answer was, in short, “No crisis, but no complacency.” We saw no evidence of a crisis, largely because the evidence of nonreproducibility and nonreplicability across all science and engineering is incomplete and difficult to assess. At the same time, steps can be taken to improve in both areas.
The committee’s specific conclusions and recommendations differed for reproducibility and replicability. One key difference involves the rates of reproducibility and replicability to which we should aspire. There is large agreement on the answer to this question for reproducibility: When a researcher transparently reports a study and makes available the underlying digital artifacts, such as data and code, the results should always be computationally reproducible. The committee made recommendations about how to achieve reproducibility, largely by improving transparency. For example, the committee proposed that, to help ensure the reproducibility of computational results, researchers should convey clear, specific, and complete information about any computational methods and data products that support their published results to enable other researchers to repeat the analysis.
The scientific ideal for replicability — in which researchers attempt to obtain consistent results by collecting new data, using similar methods — is more nuanced. For example, a key observation in the report, we believe, is that, “The goal of science is not, and ought not to be, for all results to be replicable” (p. 28), because there is a tension between replicability and discovery. (For an excellent discussion of this issue, see B. Wilson & Wixted, 2018, Advances in Methods and Practices in Psychological Science, 1, 186–197).
Similarly, the committee noted that nonreplicability can arise from a number of sources, some of which are potentially helpful to advancing scientific knowledge and others that are unhelpful.
Helpful Sources of Nonreplicability
Nonreplicability can be caused by limits in current scientific knowledge and technologies, as well as inherent but uncharacterized variabilities in the system being studied. When such nonreplicating results are investigated and resolved, it can lead to new insights, better characterization of uncertainties, and increased knowledge about the systems being studied and the methods used to study them.
Unhelpful Sources of Nonreplicability
Nonreplicability also may be due to foreseeable shortcomings in the design, conduct, and communication of a study. Whether arising from lack of understanding, perverse incentives, sloppiness, or bias, these unhelpful sources of nonreplicability reduce the efficiency of scientific progress.
One unhelpful source of nonreplicability is inappropriate statistical inference. Misuse of statistical testing often involves post hoc analysis of data already collected, making it seem as though statistically significant results provide evidence against the null hypothesis, when in fact they have a high probability of being false positives. Other inappropriate statistical practices include p-hacking — the practice of collecting, selecting, or analyzing data until a result of statistical significance is found — and “cherry picking,” in which researchers may unconsciously or deliberately selectively report their data and results.
To minimize unhelpful sources of nonreplicability, we outlined initiatives and practices to improve research design and methodology, including training in the proper use of statistical analysis and inference, improved mentoring, repeating experiments before publication, conducting rigorous peer review, utilizing tools for checking analyses and results, and improving transparency in reporting.
Replicability and reproducibility are not the only ways to gain confidence in scientific results. Research synthesis and meta-analysis can help assess the reliability and validity of bodies of research. As you probably know, meta-analyses provide estimates of overall central tendencies (effect sizes or association magnitudes), along with estimates of the variance or uncertainty in those estimates. Meta-analytic tests for variation in effect sizes can suggest potential causes of nonreplicability in existing research — in individual studies that are outliers, in particular populations, or using certain methods. Of course, such analyses must take into account the possibility that published results are biased by selective reporting and, to the extent possible, estimate its effects.
To conclude on a personal note, it was fascinating to learn about the ways that different scientific disciplines attempt to establish reproducibility and replicability. We were more convinced than ever in the fundamental soundness of our field. Like other sciences, psychological science is producing a great deal of useful and reliable knowledge — replicable discoveries about human thought, emotion, and behavior. Increasingly, researchers and governments are using such knowledge to meet social needs and solve problems, such as improving educational outcomes and reducing government waste from ineffective programs. We strongly endorse the broad conclusion from our meetings: No crisis, but no time for complacency!
Comments
Registered reports?
“The goal of science is not, and ought not to be, for all results to be replicable” (p. 28), because there is a tension between replicability and discovery.”
The first thought that came to my mind: Ostriches.
My wife, who is a Developmental Biologist with approximately 35-years of research in her CV, has to deal with replication problems in her field. And her particular field of biology is far more accurate and replicable than psychology which has built a house of cards and is wallowing in denialism!
The authors choose a loose and idiosyncratic definition of “replicability”. Nowhere does the notion that following precisely defined methods of a well designed study should yield the same results (up to the expected variability for the power of the study). Instead, there is a much looser (and barely useful) definition of addressing the same question with different data.
One consequence of this slack definition is that it makes nonreplication seem less bad. Indeed, the much remarked quote about the tension between replicability and discovery, as well as leading with “helpful sources of nonreplicability” suggests that making nonreplication seem OK might be the unstated purpose of this editorial (but thank you for telling us how you travelled to the meetings).
I find it amazing that there is no mention of pre-registration as one of the tools for minimising nonreplicability.
“The goal of science is not, and ought not to be, for all results to be replicable” (p. 28), because there is a tension between replicability and discovery.”
I’m curious – what are examples of major, verified discoveries that came from unreplicatable results and wouldn’t have been possible to come upon otherwise?
Important discoveries in the early history of electricity often failed to replicate, because of the effects of poorly understood extraneous variables such as humidity. See Heilbron, J. L. (1979). Electricity in the 17th & 18th centuries. Berkeley: University of California Press.
Reading in a scholarly early history of any field will generally reveal interesting examples of failures to replicate important findings because of unknown or poorly understood ancillary factors. In other words, at a given stage in the development of a science, one generally does not know all the factors that must in fact be controlled in order to “replicate” an earlier finding, particularly one in another lab.
More important than simple replication is building on previously obtained results, that is, doing further experiments that presuppose the earlier results.
The goal of science is not to replicate ALL results.
True and trival. New question.
How many between-subject experiments in social psychology do replicate?
Less than 25% (Science article by OSC).
Ouch.
https://replicationindex.com/2019/08/27/no-crisis-in-social-psychology/
Here’s more of the quote in context, George:
“The goal of science is not, and ought not to be, for all results to be replicable. Reports of non-replication of results can generate excitement as they may indicate possibly new phenomena and expansion of current knowledge. Also, some level of non-replicability is expected when scientists are studying new phenomena that are not well-established. As knowledge of a system or phenomenon improves, replicability of studies of that particular system or phenomenon would be expected to increase.”
Out of context, it leads to your expression of outrage. But in context, it seems more sensible than “Ostriches.”
And also, they wrote:
“The “safe” and “Bold” approaches to science have complementary advantages. One might argue that a field has become too conservative if all attempts to replicate results are successful, but it is reasonable to expect that researchers follow up on new by uncertain discovers with replications studies to sort out which promising results prove correct. Scientist should be cognizant of the level of uncertainty inherent in speculative hypotheses and in surprising results in any single study.”
@Chris Crandall. That’s an absolutely horrifying quote. Is it in the report? It doesn’t seem to be in this article. (And I’m certainly not paying to read the report.)
It’s the job of the people publishing first to make sure their result is robust. They shouldn’t be publishing stuff known to be insecure. It definitely should not be the job of others, with all the disadvantages of going second, to have to do the hard work of working out what is true.
As careful as this and other similar studies seem to be, and regardless of their conclusions, I find it astonishing that none of the examined research programs test empirically falsifiable theories about the observations, and that the absence of theories about what has been observed seems not to be a matter of comment or concern.
Evolution isn’t a cornerstone of biology because of how many observations are consistent with the idea of natural selection and how reproducible/replicable those observations are. It has that standing because it makes a testable, falsifiable theoretical statement about all such observations. In this case, we know what we know.
Dark Matter is a theory about an unknown kind of matter that exhibits behavior consistent with a universal model, measurable in precise and replicable ways, but we have no idea what it is. In this case, we know what we don’t know.
These are two of many examples of the role played by falsifiable, unifying theories in science. Some may argue that, without a theory under test, it’s not science yet, it’s observation, a precursor to science.
APS regularly opens certain online articles for discussion on our website. Effective February 2021, you must be a logged-in APS member to post comments. By posting a comment, you agree to our Community Guidelines and the display of your profile information, including your name and affiliation. Any opinions, findings, conclusions, or recommendations present in article comments are those of the writers and do not necessarily reflect the views of APS or the article’s author. For more information, please see our Community Guidelines.
Please login with your APS account to comment.