Scientists Turn to Machine Learning to Save Lives
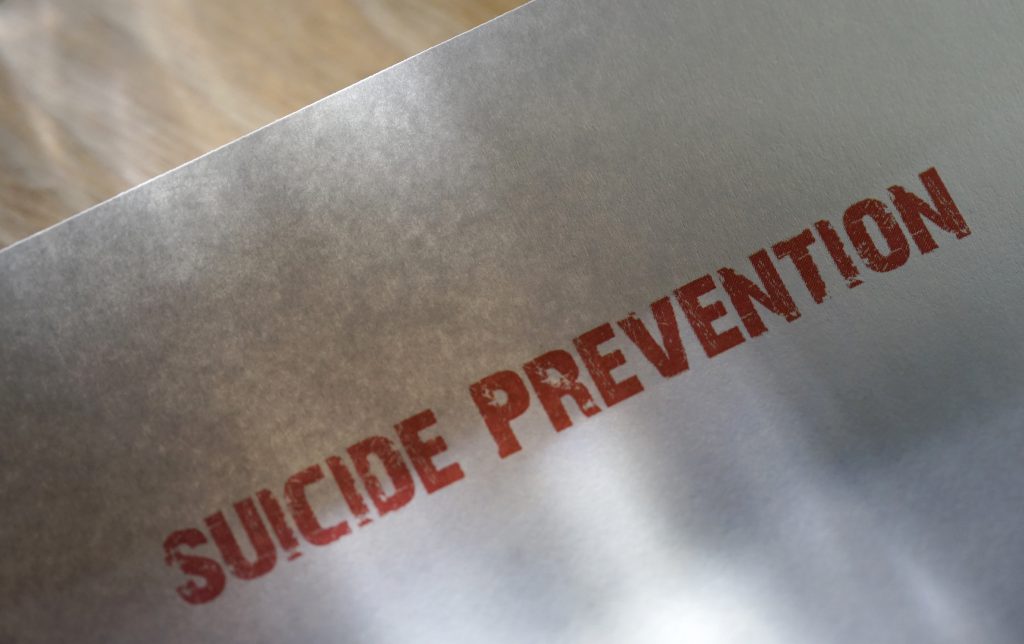
Following the suicide of a relative or close friend, surviving family members and friends are left with a number of painful questions: “What made them do it?” “Why didn’t they get help?” The most troublesome question is often, “Is there anything I could have done to prevent this?”
Clinical psychological scientists are asking that same question on a large scale and making progress on finding the answer with the use of Big Data and machine learning. Working with scientists in other disciplines including medicine and computer science, the psychological researchers hope their models will help clinicians identify and help individuals in immediate danger of dying by their own hand. Researchers are already exploring existing data sources, including medical records, brain scans, blood tests, fitness trackers, smartphones, and social media, which could be used for these models.
A Dire Need
Statistics on suicide lay bare the urgent need for better predictive models. The World Health Organization estimates that 800,000 individuals take their own lives every year, translating to about one suicide every 40 seconds. The US government’s Centers for Disease Control and Prevention recently reported a rise in suicide rates in nearly every state between 1999 and 2016. Although suicide rates on college campuses are below those in the general population, suicide is surpassed only by accidents as the leading cause of death among college students.
But research has yet to produce tools that can help clinicians predict and prevent suicides, University of Rochester psychological scientist Catherine R. Glenn and colleagues Courtney Bagge (University of Mississippi) and Andrew Littlefield (Texas Tech University) reported in a 2017 paper in Clinical Psychological Science. And most existing risk factors predict suicide ideation, but not actual suicidal behavior, they write.
“Prior studies have focused on identifying which individuals are at risk for suicidal behavior. However, much less is known about when individuals are most at risk, which is extremely important for informing clinical care (e.g., deciding whether an individual needs to be hospitalized),” Glenn and her coauthors say.
Clinicians have traditionally focused on identifying a few risk factors in populations or patients. Among military veterans, for example, suicide risk factors include post-traumatic stress disorder, opioid dosage, and having killed in war. Data indicate that men are at a higher risk for suicide than are women in all populations. But relying on only a few risk factors to assess a patient leads to a danger of false positives and false negatives.
Researchers have found that the biggest risk factor across all populations for a future suicide attempt is actually a previous attempt.
Current risk assessments generally involve lengthy interviews and questionnaires, which fall short of reliable predictive power for several reasons — including their considerable reliance on self-reports.
“To assess current suicidal thinking and potential risk for suicidal action in most clinical settings and research studies, we ask individuals to indicate if they are thinking about suicide, if they have a plan, or if they intend to act on their suicidal thoughts,” Glenn says. “People may be hesitant to respond accurately because they want to leave the hospital or do not want to be hospitalized. They may have an active suicide plan and don’t want to be stopped.”
Clinical psychological scientist David Rozek, director of training for the National Center for Veterans Studies and research fellow in psychiatry at the University of Utah, says that most assessments rely on measuring specific risk factors and on clinical judgement. But these assessments generally reveal nothing about the progression of a patient’s suicidal thoughts, making it impossible to determine whether a suicide attempt is imminent or just probable in the next year.
“Our current measures have difficulty capturing clinically meaningful change in relatively short periods of time — hours, days — as the current measures often focus on risk that is longer in duration,” Rozek said.
Predictive Algorithms
An emerging approach to developing more reliable prediction tools is the use of retrospective data in the form of electronic health records (EHRs).
“Most individuals who die by suicide will see a health-care provider in the year prior to death — and a sizeable percentage in the months and weeks prior to death,” Glenn noted. “Detection via EHR may help identify high-risk individuals in need of more intensive risk assessment and connection to mental health treatment.”
Colin Walsh, assistant professor of bioinformatics, medicine, and psychiatry at Vanderbilt University, is among the scientists developing methods to distinguish time-sensitive levels of risk. Walsh and colleagues from Florida State University combed through 5,000 patient EHRs with instances of self-injury to build a predictive algorithm for suicide attempts based on other information included in the charts. Self-injury is easy to spot from diagnostic codes in a medical chart but doesn’t always reflect a suicide attempt. So the researchers had to take a second look at each chart to find true cases of attempted suicide.
“The stakes are so high that we wanted to make sure that we really were very rigorous about the approach,” Walsh said in an interview. “We identified in this paper 5,500 charts that had [self-injury] codes in our data. Our team decided to review every single one of those charts, which is not a minor undertaking.”
Their computer program would eventually learn from this raw data how to predict suicide attempts, as the team reported in Clinical Psychological Science. Every false positive they identified before they built their model meant more accuracy in the final product.
“One of the first results we discovered was that 42 percent of the time, those codes for self-injury did not also have evidence on chart review of suicidal intent,” Walsh said.
Walsh and colleagues took those EHRs and used the data to develop a machine-learning program to find patterns. They compared records with later suicides and suicide attempts. The computer algorithm tested millions of different patterns, taking the entries in EHRs and plugging them into equations, building an accurate model through trial and error. When it was done, it could take a single medical record and calculate the probability of an individual’s attempt to kill himself. Its accuracy comes from the ability to consider so many variables and their large or small contributions to risk and to quickly solve equations. While the best human prediction models have an accuracy of about 60%, Walsh and colleagues’ algorithm identified future suicide attempts with 84% accuracy.
A Blend of Data
While Walsh and his colleagues’ model used electronic health records, machine-learning algorithms can be used with many types of data. Researchers in the United States and Canada have found differences in brain-scan and neural-response data between those who died by suicide and those who died of sudden but nonsuicide death at the same age. Military veterans who attempt suicide have distinct genetic expressions when compared with veterans who have not tried to take their own lives. These data also could be considered and built into an algorithm that uses behavioral, medical, neurological, and genomic data to make predictions.
The United States Veterans Association has already begun to incorporate predictive technology into their efforts to improve veteran well-being and prevent veteran suicides, including their Recovery Engagement and Coordination for Health-Veterans Enhanced Treatment initiative. Their efforts to identify the veterans most at risk of suicide are promising, but still in the proof-of-concept stage.
Patterns in Social Media
EHRs form a promising base for suicide risk assessment, but what about individuals who have suicidal thoughts yet never set foot in a doctor’s office or mental health facility?
Text recognition and photo analysis are opening the door to screening large swaths of the population based on
information they give up willingly, even though they might not be seeking psychological help. These algorithms scan
social-media profiles and timelines to assess a user’s state of mental health. One machine-learning algorithm was able to identify common social-media posting behaviors in military personnel who eventually killed themselves, and another spotted those later diagnosed with depression (but who did not attempt suicide) based on Instagram photo characteristics such as color saturation, brightness, and the number of faces in pictures. These patterns weren’t always distinct enough to be useful in diagnosis, but they may lead to some valuable risk-assessment tools in the future. Medical professionals or organizations that scan profiles or use social-media data in the future also will have to address privacy concerns before these data find use in clinical settings.
Into the Field
Dartmouth College psychological scientist Bill Hudenko and his partners are pushing the latest suicide risk and prevention techniques outside the lab and into the hands of clinicians. A promising 2012 study by his colleagues Rob Althoff, Sanchit Maruti, Isabelle Desjardins, and Willy Cats-Baril at the University of Vermont Medical Center indicated that a brief, adaptive questionnaire could screen emergency room (ER) patients for the risk of a suicide attempt within 72 hours as accurately as a trained psychiatrist. After partnering with Hudenko, the team found that as many as 5% of ER patients may be at high short-term risk for suicide, but only half of those patients come to the ER with a psychiatric complaint.
Hudenko saw an opportunity in the screening approach because the risk questionnaire was scored through software that was capable of increasing in predictive accuracy over time. With the novel screener as a start, Hudenko then built beyond it. His vision is a quick, easy-to-use set of questions that every ER patient in the country would fill out. Those who are flagged for high risk would be given acute care consisting of psychiatric evaluation or supervision. Once the patients leave the hospital, expert clinical help, social support, and artificial intelligence would be combined in a smartphone app to give them personal, effective care in their own environment. The app would connect the patient with a behavioral health coach, who would then link the patient with family, friends, clergy, or other people close to them who could be educated and enlisted to form a support network.
“After reviewing research on the most efficacious way to prevent suicide when someone is identified as high risk, time and again we found that one of the most important factors for maintaining safety is positive social support for the person at risk,” Hudenko said.
The patients would continue to complete risk assessments via the app, and a natural-language processor within the app could monitor their messages (with the patient’s permission) for language indicative of an imminent suicide attempt. If the app picked up on a serious risk, the behavior health coach would be able to contact the patient within 5 minutes and activate an active rescue within 10 minutes.
“One of the biggest challenges with suicide prevention is that very often those who are at greatest risk aren’t reaching out for help,” Hudenko said. “So we’re taking a different approach. We’re working to predict and understand when risk escalates so that we can reach out and prevent suicide instead of reacting to dangerous situations.”
In his new role as Chief Science Officer at Voi (a company dedicated to reducing suicide rates across the country), Hudenko and his colleagues are now researching and disseminating both the suicide-risk assessment and prevention software across the United States.
Tailoring Assessment Tools
Glenn points out that different risk-assessment strategies may be effective for different populations.
“Older adults may see their primary care doctor more often, and therefore EHR may be a richer source of information than for younger people,” she said. “For younger people, we may get a richer signal from their social media or other methods of ‘digital phenotyping’ such as active or passive monitoring via smartphone or wearable sensors.”
While these new models may not explain the mental health conditions and life circumstances that could be playing a role in an individual’s suicide risk, researchers say, the large-scale algorithms nevertheless hold the promise of identifying high-risk individuals who can be targeted for intervention or supplied with resources to voluntarily seek help.
APS regularly opens certain online articles for discussion on our website. Effective February 2021, you must be a logged-in APS member to post comments. By posting a comment, you agree to our Community Guidelines and the display of your profile information, including your name and affiliation. Any opinions, findings, conclusions, or recommendations present in article comments are those of the writers and do not necessarily reflect the views of APS or the article’s author. For more information, please see our Community Guidelines.
Please login with your APS account to comment.