Measurement Matters
After a long and cold journey of 286 days, the Mars Climate Orbiter reached its destination on 23 September 1999. Rather than beginning its mission, however, the satellite disintegrated upon entering the atmosphere because one software module made calculations in US customary units and fed them into a second module that assumed metric units. Four years later, two halves of a large bridge being constructed across the Rhine came together to connect Germany and Switzerland. To the surprise of the engineers, there was a height difference of 54 cm (21 in) between the two sides: Different measurements of sea level had been used (the North Sea vs. the Mediterranean Sea).
Measurement problems can (and do) occur — sometimes with disastrous consequences — as part of even the most remarkable scientific endeavors, such as sending a satellite into space. We are in no different a situation in psychology as we navigate the shifts in our research culture toward a more open and rigorous science. So far, these shifts have largely ignored the topic of measurement, an unfortunate situation because the quality of measurement is even more foundational than statistical practice. A high-powered, perfectly parsimonious statistical model cannot save us from poor measurement.
In psychology, measurement is especially difficult because what we want to measure often does not permit direct observation. We can directly observe the height of a person next to us on the bus, but we often have little insight into latent, psychological attributes such as intelligence, extraversion, or depression. Construct validation — showing that an instrument meant to measure a construct actually measures the construct in question — is no easy task. Not only are psychological constructs difficult to observe, they are also complex. It is relatively easy to settle on which sea should be the benchmark for calculating height above sea level, but clearly defining intelligence, extraversion, or depression is challenging. There are different ways to understand and measure these constructs because they encompass different behaviors, perceptions, subjective experiences, environmental influences, and biological predispositions.
This article highlights the neglect of psychological measurement, explains why this poses a serious and underrecognized threat to the recent replicability efforts in psychological science, and concludes with some suggestions on how to move forward.
The Problem: Neglected Measurement
To measure a psychological construct such as extraversion, psychologists often use questionnaires with multiple items. Items are added up to a score, and it is assumed that this score represents a person’s position on the construct. From “Paul has a high score on an extraversion scale,” we assume that Paul is very extroverted. This inference is not a free psychometric lunch; evidence of validity[1] is needed to support the claim. You want to have (1) a good theory supporting the items you include in your scale; (2) a scale showing acceptable psychometric properties (e.g., reliability and dimensionality); and (3) a scale related to other constructs in the ways hypothesized (e.g., convergent and discriminant validity) that captures group differences or causal processes expected to exist. Only if your scale meets these criteria can substantive inferences follow.
Unfortunately, evidence of validity is lacking in many areas of psychological research. As an example, depression is assessed in more than 1,000 research studies per year and is used as an outcome, predictor, moderator, or covariate across numerous disciplines (e.g., psychology, psychiatry, epidemiology). More than 280 different scales for assessing depression severity have been developed and used in research in the last century. Commonly used depression scales feature more than 50 different symptoms, and content overlap among scales is low. For example, one third of the symptoms in the most cited scale — the 20-item Center of Epidemiological Studies Depression scale (Radloff, 1977; approximately 41,300 citations) — do not appear in any of the other most commonly used instruments. The result is that different scales can lead to different conclusions, which has been documented many times in clinical trials. For instance, a recent clinical trial queried patients on four different scales to examine whether full-body hyperthermia was an efficacious depression treatment. The hyperthermia group showed significant improvements over placebo on only one of the four scales. Unfortunately, the authors reported the three null findings in the supplementary materials without mention in the paper. This is an important lesson: Although comparing results of multiple measures offers more robust insights, it also opens the door to p-hacking, fishing, and other questionable research practices.
There is more. Major depression had one of the lowest interrater reliabilities of all mental disorders assessed in the DSM-5 field trials, with a coefficient of 0.28, and depression scales in general are often modeled without taking into account their multidimensionality and lack of temporal measurement invariance. Similar to the case of the Orbiter, these theoretical and statistical measurement issues can have drastic consequences, biasing conclusions of research studies and introducing error into inferences — inferences that influence the real-world behavior of scientists and resource allocation in science.
Depression is not an isolated example of poor measurement practices in psychological research. Reviews within specific domains cite similar issues (e.g., emotion; Weidman, Steckler, & Tracy, 2016), and our recent work suggests that poor practices span topics and subdisciplines. In a systematic review of a representative sample of 35 empirical articles published in the Journal of Personality and Social Psychology in 2014, we identified 433 scales aimed to measure psychological constructs. Of these, about half contained no citation to any validation study. For many scales, Cronbach’s alpha was the sole psychometric property, and for one in five scales, no psychometric information whatsoever was reported. Simplified, evidence of validity, in practice, forms a hierarchy: (1) none, (2) alpha only, (3) a citation, presumably to another paper that contains validity evidence, and (4) more evidence, which takes a variety of forms. Further, we saw signs of researcher degrees of freedom, similar to the depression literature: Authors used multiple scales to measure one construct without justifying their use of a particular scale. We also noted that scale modification (adding or removing items) was common, as was combining multiple scales to a single index without a transparent rationale.
Poor Measurement Complicates Replications
Taking the results of these studies together, it is difficult to ignore the connection between poor measurement practices and current discussions about replicability. For example, Monin, Sawyer, and Marquez (2008) used a variety of scales in their study, which were also administered in the replication study as a part of the “Reproducibility Project: Psychology.” However, the replication study identified different factor solutions in the primary measures, indicating that different items formed different factors. How are we to interpret the result of this study? Is it a theory failure, a replication failure, or a measurement failure? Again, these questions hold broadly. For depression, for instance, the factor structure of a given scale often differs across samples, across time in the same sample, and even in large subsets of the same sample.
If a scale lacks validity or measures different constructs across samples, there is little benefit in conducting replication studies. We must take a step back and discern how to define and measure the variables of interest in the first place. In such cases, what we need are validity studies, not replication studies. Our work to promote replicability in psychology will be stymied absent improving our measurement practices. Making replications mainstream must go hand in hand with making measurement theory mainstream.
Ways Forward
Norms are changing in psychology, and recent articles and publisher policies push psychological scientists toward more rigorous and open practices. However, contributions focusing on the connection between measurement and replicability remain scant. We therefore close with some nontechnical suggestions that we hope will be relevant to researchers from all subdisciplines of psychology.
Clearly communicate the construct you aim to measure, how you define the construct, how you measure it, and the source of the measure.
Provide a rationale when using a specific scale over others or when modifying a scale. If possible, use multiple measures to demonstrate either robust evidence for a finding or the sensitivity of a finding to particular scales.
Preregister your study. This counters selective reporting of favorable outcomes, exploratory modifications of measures to obtain desired results, and overinterpretation of inconclusive findings across measures.
Consider the measures you use in your research. What category of validity evidence (none, alpha, citation, or more) would characterize them? If your measures fall into the first two categories, consider conducting a validation study (examples are provided below). If you cannot do so, acknowledge measurement as a limitation of your research.
Stop using Cronbach’s alpha as a sole source of validity evidence. Alpha’s considerable limitations have been acknowledged and clearly described many times (e.g., Sijtsma, 2009). Alpha cannot stand alone in describing a scale’s validity.
Take the above points into consideration when reviewing manuscripts for journals or when serving as an editor. Ensure authors report the necessary information regarding the measurement so that readers can evaluate and replicate the measurement in follow-up studies, and help change the measurement standards of journals you work for.
We recognize that measurement research is difficult. Measurement requires both theoretical and methodological expertise. Good psychometric practice cannot make up for a poorly defined construct, and a well-defined construct cannot make up for poor psychometrics. For those reasons, it is hard to come up with a few quick fixes to improve measurement. Instead, we recognize that many psychologists may not have had training in validity theory or psychometrics and provide a list of resources for those interested in learning more. These include a collection of seminal materials on measurement and validation, as well as some accessible examples.
In closing, we want to share the screenshot of the Wikipedia article on Psychological Measurement (see Figure 1), which auto-directs to the page for Psychological Evaluation.
We couldn’t agree more: Measurement deserves more attention.
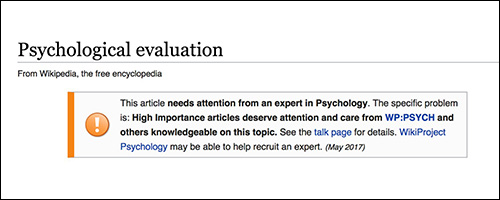
Figure 1. This screenshot of the Wikipedia article on Psychological Measurement auto-directs to the page for Psychological Evaluation.
The authors would like to thank Jolynn Pek, Ian Davidson, and Octavia Wong for their ongoing work in forming some of the ideas presented here.
1 We acknowledge the old and ongoing philosophical debate about how to best define validity and measurement in psychology. A detailed discussion of validity theory is beyond the scope of this article and is described at length elsewhere (e.g., American Educational Research Association, American Psychological Association, & National Council on Measurement in Education, 2014; Borsboom, Mellenbergh, & van Heerden, 2004; Kane, 2013). Here, we discuss validity consistent with Loevinger’s (1957) seminal work on construct validation.
References and Further Reading
Aiken, L. S., West, S. G., & Millsap, R. E. (2008). Doctoral training in statistics, measurement, and methodology in psychology: Replication and extension of Aiken, West, Sechrest, and Reno’s (1990) survey of PhD programs in North America. American Psychologist, 63, 32–50. doi:10.1037/0003-066X.63.1.32
American Educational Research Association, American Psychological Association, & National Council on Measurement in Education. (2014). Standards for educational and psychological testing. Washington, DC: Joint Committee on Standards for Educational and Psychological Testing.
Borsboom, D., Mellenbergh, G. J., & van Heerden, J. (2004). The concept of validity. Psychological Review, 111, 1061–1071. doi:10.1037/0033-295X.111.4.1061
Flake, J. K., Pek, J., & Hehman, E. (2017). Construct validation in social and personality research: Current practice and recommendations. Social Psychological and Personality Science, 8, 370–378.
Fried, E. I. (2017). The 52 symptoms of major depression. Journal of Affective Disorders, 208, 191–197. doi:10.1016/j.jad.2016.10.019
Fried, E. I., & Nesse, R. M. (2015). Depression is not a consistent syndrome: An investigation of unique symptom patterns in the STAR*D study. Journal of Affective Disorders, 172, 96–102. doi:10.1016/j.jad.2014.10.010
Fried, E. I., van Borkulo, C. D., Epskamp, S., Schoevers, R. A., Tuerlinckx, F., & Borsboom, D. (2016). Measuring depression over time . . . or not? Lack of unidimensionality and longitudinal measurement invariance in four common rating scales of depression. Psychological Assessment, 28, 1354–1367. doi:10.1037/pas0000275
Janssen, C. W., Lowry, C. A., Mehl, M. R., Allen, J. J. B., Kelly, K. L., Gartner, D. E., … Raison, C. L. (2016). Whole-body hyperthermia for the treatment of Major Depressive Disorder. JAMA Psychiatry, 53706, 1–7. doi10.1001/jamapsychiatry.2016.1031
Kane, M. T. (2013). Validating the interpretations and uses of test scores. Journal of Educational Measurement, 50, 1–73. doi:10.1111/jedm.12000
Loevinger, J. (1957). Objective tests as instruments of psychological theory. Psychological Reports, 3, 635–694.
Monin, B., Sawyer, P. J., & Marquez, M. J. (2008). The rejection of moral rebels: Resenting those who do the right thing. Journal of Personality and Social Psychology, 95, 76–93. doi:10.1037/0022-3514.95.1.76
Open Science Collaboration. (2015). Estimating the reproducibility of psychological science. Science, 349, aac4716-aac4716. doi:10.1126/science.aac4716
Radloff, L. S. (1977). The CES-D scale: A self-report depression scale for research in the general population. Applied Psychological Measurement, 1, 385–401. doi:10.1177/014662167700100306
Regier, D. A., Narrow, W. E., Clarke, D. E., Kraemer, H. C., Kuramoto, S. J., Kuhl, E. A., & Kupfer, D. J. (2013). DSM-5 field trials in the United States and Canada, part II: Test-retest reliability of selected categorical diagnoses. The American Journal of Psychiatry, 170, 59–70. doi:10.1176/appi.ajp.2012.12070999
Santor, D. A., Gregus, M., & Welch, A. (2006). Eight decades of measurement in depression. Measurement, 4, 135–155. doi:10.1207/s15366359mea0403
Sijtsma, K. (2009). On the use, the misuse, and the very limited usefulness of Cronbach. Psychometrika, 74, 107–120. doi:10.1007/s11336-008-9101-0
Weidman, A. C., Steckler, C. M., & Tracy, J. L. (2017). The jingle and jangle of emotion assessment: Imprecise measurement, casual scale usage, and conceptual fuzziness in emotion research. Emotion, 17, 267–295.
Zwaan, R. A., Etz, A., Lucas, R. E., & Donnellan, M. B. (2018). Making replication mainstream. Behavioral and Brain Sciences. Advance online publication. doi:10.1017/S0140525X17001972
Comments
It would be nice if there were a list of references for the citations in the article.
Hi Mary, there is a reference list at the end of the article (at least when I view the article in my browser). For further references, see our reading list:
https://docs.google.com/document/d/11jyoXtO0m2lUywpC04KjLvI5QcBUY4YtwEvw6cg2cMs/
The comments about Cronbach’s Alpha as a source of information about validity are puzzling: Alpha is an index of internal-consistency reliability.
Among other resources, Cronbach & Meehl (1955) discussed how reliability is an aspect of construct validity.
The Denman Effect
Christopher Denman drops in
Punctually once a year,
Yet surprised I always am
Him earlier to behold.
This anomaly in time
I will name, ‘ The Denman Effect ‘,
For Denman shows me that time
Flows faster as one grows old!
Boghos L. Artinian
This is not an article about measurement, but about sets of assumption-laden pragmatic ‘procedures’ which enable some kind of systematic psychological assessment of non-quantitative psychological attributes.
While I am sympathetic to the authors’ desire to address measurement issues in psychology, especially in the context of replication and replicability, ignoring the now substantive literature on these topics is hardly the way to proceed.
There are many ways to establish reliability and validity of an assessment that use methods and reasoning that might be found within any science in which the attributes in question lack any evidence for their quantitative structure.
Perhaps a close reading of:
Barrett, P.T. (2018). The EFPA test-review model: When good intentions meet a methodological thought disorder. Behavioural Sciences (https://www.mdpi.com/2076-328X/8/1/5), 8,1, 5, 1-22. [open-access] might assist as this contains 60 or so references to the major publications on measurement issues over the last 20+ years. It also outlines the now delicate legal situation for those presenting psychometric indices/latent variable models etc. to a court of law as though these are ‘evidence’ for anything in particular.
More specifically Joel Michell, Mike Maraun, and Gunter Trendler have written extensively on the constituent properties of measurement, with Gunter addressing the replicability problem expected when psychologists pretend to measure attributes as quantities.
And James Grice at OSU has now provided psychologists with a means to undertake powerful causal modeling in psychology without needing to make unreal and untenable assumptions (his free-to-use Observation Oriented Modeling software and methodology).
Some Key References
Grice, J. (2011). Observation Oriented Modeling: Analysis of cause in the behavioral sciences. New York: Academic Press. ISBN: 978-012-385194-9.
The software and other information, including videos, manuals etc. can be downloaded from:
http://www.idiogrid.com/OOM/
Grice, J. (2015). From means and variances to persons and patterns. Frontiers in Psychology: Quantitative Psychology and Measurement (http://dx.doi.org/10.3389/fpsyg.2015.01007 ), 6:1007, , 1-12.
Grice, J., Barrett, P., Cota, L., Felix, C., Taylor, Z., Garner, S., Medellin, E., & Vest, A. (2017). Four bad habits of modern psychologists. Behavioural Sciences (http://www.mdpi.com/2076-328X/7/3/53), 7(3), 53, 1-21.
Michell, J. (1997). Quantitative science and the definition of measurement in Psychology. British Journal of Psychology, 88, 3, 355-383.
Michell, J. (2008). Is Psychometrics Pathological Science?. Measurement: Interdisciplinary Research & Perspective, 6, 1, 7-24.
Michell, J. (2009). Invalidity in Validity. In Lissitz, R.W. (Eds.). The Concept of Validity: Revisions, New Directions, and Applications (Chapter 6, pp. 111-133). Charlotte, NC: Information Age Publishing. ISBN: 9781-60752-227-0.
Michell, J. (2012). Alfred Binet and the concept of heterogeneous orders. Download link:
http://www.frontiersin.org/quantitative_psychology_and_measurement/10.3389/fpsyg.2012.00261/abstract. Frontiers in Quantitative Psychology and Measurement, 3, 261, 1-8.
Maraun, M.D. (1998). Measurement as a normative practice: Implications of Wittgenstein’s philosophy for measurement in Psychology. Theory & Psychology, 8, 4, 435-461.
Maraun, M.D. (2007). Myths and Confusions. http://www.sfu.ca/~maraun/myths-and-confusions.html, , , 0-0.(open-access book on the myth of latent variables in psychology)
Trendler, G. (2009). Measurement Theory, Psychology and the Revolution That Cannot Happen. Theory and Psychology, 19, 5, 579-599.
Trendler, G. (2013). Measurement in psychology: A case of ignoramus et ignorabimus? A rejoinder. Theory and Psychology, 23, 5, 591-615.
Trendler, G. (2018). Conjoint measurement undone. Theory and Psychology (http://journals.sagepub.com/doi/abs/10.1177/0959354318788729), In Press, , 1-29.
Construct validity as a sensible concept died a death back in 2009, along with that other old chestnut “nomological net”:
Borsboom, D., Cramer, A.O.J., Kievit, R.A., Scholten, A.Z., & Franic, S. (2009). The end of construct validity. In Lissitz, R.W. (Eds.). The Concept of Validity: Revisions, New Directions, and Applications (Chapter 7, pp. 135-170). Charlotte, NC: Information Age Publishing. ISBN: 9781-60752-227-0.
I can’t speak for the two authors but perhaps a reply to the central point you make can be found here: https://vimeo.com/256145513; at 39.30, talking about classical measurement Denny Borsboom says ‘this is Joel Michell, he’s a very nice man actually and great scholar although I totally disagree with him , well that’s science.’ He says Michell’s position is an extreme one and that’s why he disagrees with him. You can have a listen but I fear you won’t hear any serious argument/rationale for him not agreeing with Michell!
APS regularly opens certain online articles for discussion on our website. Effective February 2021, you must be a logged-in APS member to post comments. By posting a comment, you agree to our Community Guidelines and the display of your profile information, including your name and affiliation. Any opinions, findings, conclusions, or recommendations present in article comments are those of the writers and do not necessarily reflect the views of APS or the article’s author. For more information, please see our Community Guidelines.
Please login with your APS account to comment.