Machine Learning Might Help Identify Those Most At Risk of Suicide
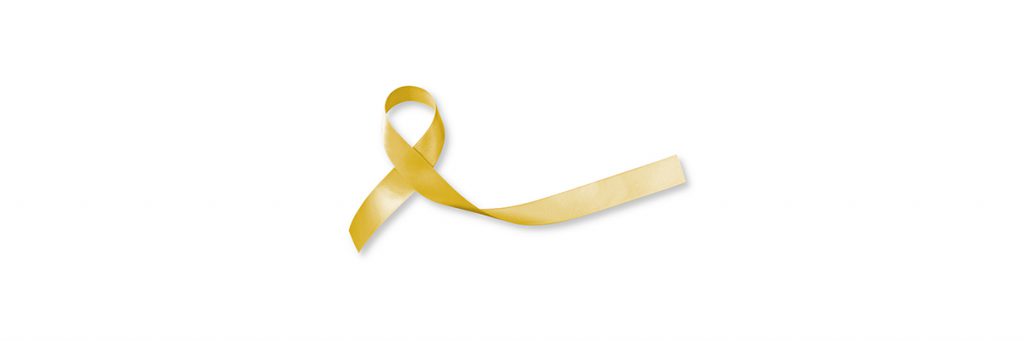
Suicide attempts are a leading public health issue worldwide, with an estimated 25 million nonfatal suicide attempts occurring every year. In the United States alone, an average of 120 people die by suicide every day. An integrative team of researchers from Vanderbilt University Medical Center and Florida State University is testing a new – and hopefully more effective way – to identify key risk factors for suicidal behavior.
Colin G. Walsh, Jessica D. Ribeiro, and Joseph C. Franklin are hoping to prevent suicides by harnessing the computing power of machine learning and artificial intelligence.
Machine learning is a form of artificial intelligence focused on developing algorithms that have the ability to “learn” without being explicitly programmed. Rather than following a set of static rules, machine learning algorithms can adapt in response to new data. This dynamism is what has allowed computers to win Jeopardy! and beat chess masters.
“Although machine learning has been a part of the computer science field for many decades, it has only recently been applied to clinical psychology,” Walsh and colleagues write in Clinical Psychological Science.
Meta-analyses on hundreds of studies reveal a disappointing trend in our ability to predict suicidal behavior: It hasn’t improved beyond random chance in over 50 years.
“The primary reason for this lack of progress is that researchers have almost always used a single factor (i.e., a simple algorithm) to predict future suicide attempts,” the researchers write. “Fortunately, [machine learning] represents a potentially effective approach for the development of complex algorithms capable of solving (or making substantial progress toward solving) complex classification problems.”
One reason for this lack of progress, according to Walsh and colleagues, is that researchers have almost always relied on a single factor (e.g., a depression diagnosis) to predict future suicide attempts. Machine learning on the other hand, can test a wide range of intricate associations among hundreds of potential factors to optimize prediction.
“Traditional statistical techniques are not ideal for such analyses; fortunately, machine learning techniques are well suited for such problems,” the research team explains.
For their experiment, Walsh and colleagues used a massive data repository containing the electronic health records of about 2 million de-identified Tennessee hospital patients. The electronic health records included a wealth of data points including, age, gender, race/ethnicity, prior medical diagnoses, prior hospitalization, prior suicide attempts, socioeconomic status, zip code, and medication prescriptions.
By carefully sifting through this data, the researchers were able to use insurance claim codes (i.e., ICD-9) to identify 5,167 patients with a record of self-injury. Experts reviewed these records and determined that 3,250 of these patients had made a suicide attempt. The other 1,917 patients comprised a comparison group where health records suggested self-injury but not a suicide attempt. These individuals typically received these codes for unintentional drug overdoses, accidental injury, non-suicidal self-injury, or injury with unclear intent.
The research team then developed different sets of machine learning algorithms with information from 1 week to 720 days before the suicide attempt. This would allow them to see whether certain factors became more or less important to making accurate predictions as the suicide attempt became more imminent (i.e., from 720 to 7 days before the attempt).
The machine learning algorithms were far more accurate at predicting suicidal behavior than traditional methods using linear regression. While linear regression might reach up to 60% accuracy, the machine learning algorithms were accurate around 80% of the time – and these models steadily improved as the suicide attempt become more imminent.
“This study provides evidence that we can predict suicide attempts accurately,” Ribeiro said in a press release. “We can predict them accurately over time, but we’re best at predicting them closer to the event. We also know, based on this study, that risk factors — how they work and how important they are — also change over time.”
Some predictors were consistently important for prediction accuracy (e.g., psychotic disorders and recurrent depression), while other factors were important only in the days or months immediately preceding the suicide attempt (e.g., age, certain substance use diagnoses, prescription for melatonin receptor agonists).
However, the researchers emphasize that although this research is promising, these analyses were exploratory and these findings should be regarded as “tentative, associative, and exploratory.”
Studies show about 60-90% of people who die by suicide had visited their medical provider within the previous year. In the future, these machine learning algorithms could be used as a kind of alert system to help clinicians accurately identify patients at heightened risk of suicidal behavior.
“This algorithm funnels our attention to the folks who are most likely to attempt suicide so our resources are better devoted to people we’re missing now,” Ribeiro said. “Right now, we’re missing a large proportion of people who are at risk that we never even think about.”
Reference
Walsh, C. G., Ribeiro, J. D., & Franklin, J. C. (2017). Predicting Risk of Suicide Attempts Over Time Through Machine Learning. Clinical Psychological Science. doi: 10.1177/2167702617691560
Comments
I would have really appreciated knowing the specifics or at least 2-3 examples of the predictors so that I could, possibly, apply them in my practice.
APS regularly opens certain online articles for discussion on our website. Effective February 2021, you must be a logged-in APS member to post comments. By posting a comment, you agree to our Community Guidelines and the display of your profile information, including your name and affiliation. Any opinions, findings, conclusions, or recommendations present in article comments are those of the writers and do not necessarily reflect the views of APS or the article’s author. For more information, please see our Community Guidelines.
Please login with your APS account to comment.